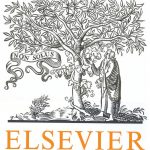
ترجمه مقاله نقش ضروری ارتباطات 6G با چشم انداز صنعت 4.0
- مبلغ: ۸۶,۰۰۰ تومان
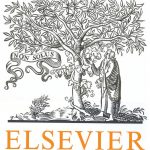
ترجمه مقاله پایداری توسعه شهری، تعدیل ساختار صنعتی و کارایی کاربری زمین
- مبلغ: ۹۱,۰۰۰ تومان
ABSTRACT
We propose and analyze a novel framework for learning sparse representations based on two statistical techniques: kernel smoothing and marginal regression. The proposed approach provides a flexible framework for incorporating feature similarity or temporal information present in data sets via non-parametric kernel smoothing. We provide generalization bounds for dictionary learning using smooth sparse coding and show how the sample complexity depends on the L1 norm of kernel function used. Furthermore, we propose using marginal regression for obtaining sparse codes which significantly improves the speed and allows one to scale to large dictionary sizes easily. We demonstrate the advantages of the proposed approach, both in terms of accuracy and speed by extensive experimentation on several real data sets. In addition, we demonstrate how the proposed approach can be used for improving semi-supervised sparse coding.
8. Discussion and future work
We propose a simple framework for incorporating similarity in feature space and space or time into sparse coding. We also propose in this paper modifying sparse coding by replacing the lasso optimization stage by marginal regression and adding a constraint to enforce incoherent dictionaries. The resulting algorithm is significantly faster. This facilitates scaling up the sparse coding framework to large dictionaries, an area which is usually restricted due to intractable computation. This work leads to several interesting follow-up directions. On the theoretical side, local convergence of Lasso-based sparse coding has been analyzed recently – preliminary examination suggests that the proposed marginal-regression based sparse coding algorithm might be more favorable for the local convergence analysis. It is also interesting to explore tighter generalization error bounds by directly analyzing the solutions of the marginal regression based iterative algorithm. Methodologically, it is interesting to explore if using an adaptive or non-constant kernel bandwidth leads to higher accuracy. Furthermore alternative methods for imposing incoherence constraints that may lead to easier optimization is an interesting direction to investigate.