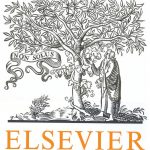
ترجمه مقاله نقش ضروری ارتباطات 6G با چشم انداز صنعت 4.0
- مبلغ: ۸۶,۰۰۰ تومان
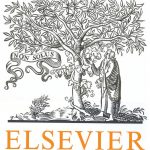
ترجمه مقاله پایداری توسعه شهری، تعدیل ساختار صنعتی و کارایی کاربری زمین
- مبلغ: ۹۱,۰۰۰ تومان
Abstract
With the ever increasing number of applications of data mining, high-utility itemset mining (HUIM) has become a critical issue in recent decades. In traditional HUIM, the utility of an itemset is defined as the sum of the utilities of its items, in transactions where it appears. An important problem with this definition is that it does not take itemset length into account. Because the utility of larger itemset is generally greater than the utility of smaller itemset, traditional HUIM algorithms tend to be biased toward finding a set of large itemsets. Thus, this definition is not a fair measurement of utility. To provide a better assessment of each itemset’s utility, the task of high average-utility itemset mining (HAUIM) was proposed. It introduces the average utility measure, which considers both the length of itemsets and their utilities, and is thus more appropriate in real-world situations. Several algorithms have been designed for this task. They can be generally categorized as either level-wise or pattern-growth approaches. Both of them require, however, the amount of computation to find the actual high average-utility itemsets (HAUIs). In this paper, we present an efficient average-utility (AU)-list structure to discover the HAUIs more efficiently. A depth-first search algorithm named HAUI-Miner is proposed to explore the search space without candidate generation, and an efficient pruning strategy is developed to reduce the search space and speed up the mining process. Extensive experiments are conducted to compare the performance of HAUI-Miner with the state-of-the-art HAUIM algorithms in terms of runtime, number of determining nodes, memory usage and scalability.
7. Conclusion and future work
Traditional high-utility itemset mining (HUIM) considers purchase quantities and unit profits of items to discover high-utility itemsets (HUIs). Because the utility of larger itemset is generally greater than the utility of smaller itemset, traditional HUIM algorithms tend to be biased toward finding large itemsets. Thus, the traditional utility measure is not a fair measurement in realworld applications. To address this issue, the problem of high average-utility itemset mining (HAUIM) has been proposed. HAUIM has attracted a lot of attention since it provides a useful alternative interestingness measure to evaluate the discovered patterns. In this paper, an efficient average-utility (AU)-list structure is designed to store the information needed to discover HAUIs. The HAUI-Miner algorithm discovers HAUIs by exploring a set-enumeration tree using a depth-first search. An efficient pruning strategy is also developed to prune unpromising candidates early and thus reduce the search space. Substantial experiments were conducted on both real-life and synthetic datasets to evaluate the efficiency and effectiveness of the designed algorithm in terms of runtime, number of determining nodes, memory consumption usage, and scalability. Performance was compared with the state-of-the-art HAUP-growth, PAI and HAUI-Tree algorithms. In this paper, the HAUI-Miner algorithm was designed to discover HAUIs efficiently in a static database. However, in real-life situations, transactions are frequently updated. New transactions may be frequently added to the original database. In future work, we will thus consider developing several algorithms to mine HAUIs in incremental databases and in data streams. Besides, with the rapid growth of information technology, it is also a critical issue to mine HAUIs in big data.