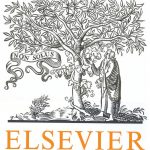
ترجمه مقاله نقش ضروری ارتباطات 6G با چشم انداز صنعت 4.0
- مبلغ: ۸۶,۰۰۰ تومان
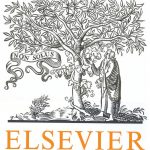
ترجمه مقاله پایداری توسعه شهری، تعدیل ساختار صنعتی و کارایی کاربری زمین
- مبلغ: ۹۱,۰۰۰ تومان
Abstract
Solar cells that convert sunlight into electrical energy are the main component of a solar power system. Quality inspection of solar cells ensures high energy conversion efficiency of the product. The surface of a multi-crystal solar wafer shows multiple crystal grains of random shapes and sizes. It creates an inhomogeneous texture in the surface, and makes the defect inspection task extremely difficult. This paper proposes an automatic defect detection scheme based on Haar-like feature extraction and a new clustering technique. Only defect-free images are used as training samples. In the training process, a binary-tree clustering method is proposed to partition defect-free samples that involve tens of groups. A uniformity measure based on principal component analysis is evaluated for each cluster. In each partition level, the current cluster with the worst uniformity of inter-sample distances is separated into two new clusters using the Fuzzy C-means. In the inspection process, the distance from a test data point to each individual cluster centroid is computed to measure the evidence of a defect. Experimental results have shown that the proposed method is effective and efficient to detect various defects in solar cells. It has shown a very good detection rate, and the computation time is only 0.1 s for a 550 × 550 image.
4. Conclusions
This paper has proposed a machine vision method for solar cell inspection in electroluminescence images. The Haar-like features are designed and extracted to represent the characteristics of local crystal-grain patterns. The improved clustering procedure can effectively group a dataset containing tens of clusters by evaluating the uniformity of inter-sample distances in each cluster. In the training process, only defect-free images are taken as training samples, and a simple distance threshold is automatically determined for each cluster. The adaptive distance threshold is easily determined based on the mean and standard deviation of distances from sample points to the cluster centroid for each individual cluster. In the inspection process, the distance from the testing data to individual cluster centroids can be easily computed and compared with the distance threshold. The proposed method effectively detects solar cell defects in electroluminescence images, and gives the location of a detected defect. With the implementation of integral image techniques, the computation time in the inspection process is extremely fast. The proposed method is thus practical for one-line, real-time inspection in the solar cell manufacturing process. The proposed method mainly focuses on the detection of lineand bar-type defects, including crack, break and finger interruption, which are serious and commonly-occurred in the solar cell manufacturing process. Other types of defects, such as circularshaped stains and contaminations in solar cells, may need different discriminative features for better inspection results. But, the proposed clustering mechanism can be still applied for the grouping of defect-free samples.