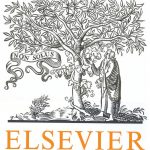
ترجمه مقاله نقش ضروری ارتباطات 6G با چشم انداز صنعت 4.0
- مبلغ: ۸۶,۰۰۰ تومان
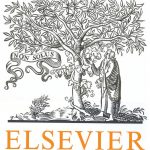
ترجمه مقاله پایداری توسعه شهری، تعدیل ساختار صنعتی و کارایی کاربری زمین
- مبلغ: ۹۱,۰۰۰ تومان
Abstract
We introduce a novel, efficient methodology for the automatic recognition of major vertical displacements in human activities. It is based exclusively on barometric pressure measured by sensors commonly available on smartphones and tablets. We evaluate various algorithms to distinguish dynamic activities, identifying four different categories: standing/walking on the same floor, climbing stairs, riding an elevator and riding a cable-car. Activities are classified using standard deviation and slope of barometric pressure. We leverage three different inference models to predict the action performed by a user, namely: Bayesian networks, decision trees, and recurrent neural networks. We find that the best results are achieved with a recurrent neural network (reaching an overall error rate of less than 1%). We also show that decision tree classifiers can achieve good accuracy and offer a better trade-off between computational overhead and energy consumption; therefore, they are good candidates for smartphone implementations. As a proof of concept, we integrate the decision tree classifier in an App that infers user activity and measures elevation differences. Test results with various users show an average recognition accuracy rate of about 95%. We further show the power consumption of running barometric pressure measurements and analyse the correlation of pressure with environmental factors. Finally, we compare our approach to other standard methodologies for activity detection based on accelerometer and/or on GPS data. Our results show that our technique achieves similar accuracy while offering superior energy efficiency, independence from the sensor location, and immunity to environmental factors (e.g., weather conditions, air handlers).
8. Conclusion
The barometer is one of the least frequently used sensors on smartphones, but is also one of the most promising. In this work, we demonstrated the advantages of the use of barometric pressure data from sensors embedded in mobile phones to recognize verti- cal displacement activities. We evaluated the performance of three different models to infer user activities and found that the LSTM recurrent neural network has a very high accuracy rate. How- ever, J48 decision tree algorithm is a good choice for resource- constrained devices owing to its fairly high accuracy, its low com- putational overhead, and (consequently) its low energy consump- tion. We implemented an Android application that integrates the J48 decision tree algorithm and infers the activity performed by a user. The application uses barometric pressure data to provide information on the vertical distance travelled by a user and also shows it instantaneously on a graph. We also showed that baro- metric pressure sensors have many advantages over sensors tradi- tionally used for activity recognition (namely, accelerometers and GPS) in terms of accuracy, energy efficiency, indoor effectiveness and independence from the phone position. The use of baromet- ric data for activity recognition is very advantageous, as many ap- plications that cannot be correctly recognized with accelerometer can be easily inferred with pressure data. Furthermore, the baro- metric sensor can enhance the quality of accelerometer sampling whenever a vertical displacement is present but is not the main movement. Finally, the barometer can also be used as a trigger to accelerometer sensing when the barometer itself cannot achieve a sufficient quality, resulting in a more power-efficient approach. Future work includes the use of multiple sensors for activity detection and the implementation of a mechanism for switching between sensors – and their underlying methodology – depending on geo-position, predominant activity, and objective functions like e.g., battery life and accuracy optimization.