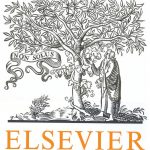
ترجمه مقاله نقش ضروری ارتباطات 6G با چشم انداز صنعت 4.0
- مبلغ: ۸۶,۰۰۰ تومان
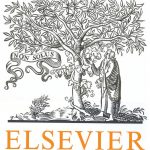
ترجمه مقاله پایداری توسعه شهری، تعدیل ساختار صنعتی و کارایی کاربری زمین
- مبلغ: ۹۱,۰۰۰ تومان
abstract
In this work, the modification of the probabilistic neural network (PNN) is proposed. The traditional network is adjusted by introducing the weight coefficients between pattern and summation layer. The weights are derived using the sensitivity analysis (SA) procedure. The performance of the weighted PNN (WPNN) is examined in data classification problems on benchmark data sets. The obtained WPNN’s efficiency results are compared with these achieved by a modified PNN model put forward in literature, the original PNN and selected state-of-the-art classification algorithms: support vector machine, multilayer perceptron, radial basis function neural network, k-nearest neighbor method and gene expression programming algorithm. All classifiers are collated by computing the prediction accuracy obtained with the use of a k-fold cross validation procedure. It is shown that in seven out of ten classification cases, WPNN outperforms both the weighted PNN classifier introduced in literature and the original model. Furthermore, according to the ranking statistics, the proposed WPNN takes the first place among all tested algorithms.
6. Conclusions
In this paper, the weighted probabilistic neural network was proposed. The original PNN model was enriched by the weights introduced between pattern and summation layer of the network. The analytical formula for the weighting coefficients was provided with the use of the SA procedure. A 10-fold cross validation accuracy of WPNN was compared with the accuracy of the modified PNN available in literature, the traditional PNN and the state-of-the-art algorithms in ten benchmark data classification problems. Also, the ranking points statistics were calculated along with Friedman test which confirmed the consistency of the comparison. The elements of novelty of this study are as follows:
• The strict analytical formula for the weights of PNN has not been provided up to this date. In this work, we propose such a formula. It stems from the SA procedure, therefore is interpretable and easy to implement.
• Due to introduced weights, neither heuristic nor approximate but precise results are obtained.
• SA procedure has not been utilized for computing the PNN’s weights so far.
• The computation of weights is strictly embedded in the operation of PNN. Therefore, no additional iterative algorithm which relies on the minimization of assumed error function is required.
• From the effectiveness point of view, the proposed WPNN is not predominant in comparison to other approaches. However, our greatest attention in this study is paid to the fact of improving the prediction ability of WPNN with respect to the modified model proposed in literature and original network. This goal is achieved in majority of classification problems since WPNN attains higher accuracy than MPNN original PNN in seven out of ten considered tasks. Moreover, in single data classification case, WPNN’s accuracy is only less than 0.5% lower in contrast to other PNN based methods. This outcome makes here all the networks almost identical in terms of performance. Furthermore, if we consider the total ranking points as the comparative statistics, WPNN stacks up much better against the other models