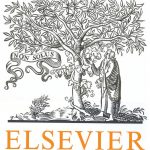
ترجمه مقاله نقش ضروری ارتباطات 6G با چشم انداز صنعت 4.0
- مبلغ: ۸۶,۰۰۰ تومان
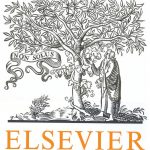
ترجمه مقاله پایداری توسعه شهری، تعدیل ساختار صنعتی و کارایی کاربری زمین
- مبلغ: ۹۱,۰۰۰ تومان
Abstract
Wide spread monitoring cameras on construction sites provide large amount of information for construction management. The emerging of computer vision and machine learning technologies enables automated recognition of construction activities from videos. As the executors of construction, the activities of construction workers have strong impact on productivity and progress. Compared to machine work, manual work is more subjective and may differ largely in operation flow and productivity among different individuals. Hence only a handful of work studies on vision based action recognition of construction workers. Lacking of publicly available datasets is one of the main reasons that currently hinder advancement. The paper studies worker actions comprehensively, abstracts 11 common types of actions from 5 kinds of trades and establishes a new real world video dataset with 1176 instances. For action recognition, a cutting-edge video description method, dense trajectories, has been applied. Support vector machines are integrated with a bag-of-features pipeline for action learning and classification. Performances on multiple types of descriptors (Histograms of Oriented Gradients – HOG, Histograms of Optical Flow – HOF, Motion Boundary Histogram – MBH) and their combination have been evaluated. Discussion on different parameter settings and comparison to the state-of-the-art method are provided. Experimental results show that the system with codebook size 500 and MBH descriptor has achieved an average accuracy of 59% for worker action recognition, outperforming the state-of-the-art result by 24%.
6. Conclusions
In this paper, we studied vision-based worker action recognition using the Bag-of-Feature framework. A cutting-edge video representation method – dense trajectories was adopted. Three types of descriptors, namely HoG, HoF and MBH, and their combination were tested for performance evaluation. The multi-class SVM with non-linear RBF kernel was applied for training and classification. A new real world dataset were established for system validation with totally 1176 video clips, covering 11 categories of common worker actions. Several challenging situations, such as view angle change, illumination change, interrupted workflow, and interaction between multiple workers, are involved in the proposed dataset. Experimental results showed that the system achieved the best performance with average accuracy of 59% under the configuration of MBH descriptor and codebook size 500, outperforming Gong et al.’s method [43] by 24%. The system holds a promising potential for future real world application