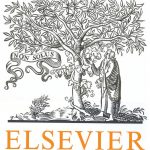
ترجمه مقاله نقش ضروری ارتباطات 6G با چشم انداز صنعت 4.0
- مبلغ: ۸۶,۰۰۰ تومان
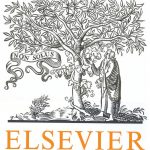
ترجمه مقاله پایداری توسعه شهری، تعدیل ساختار صنعتی و کارایی کاربری زمین
- مبلغ: ۹۱,۰۰۰ تومان
Abstract
Materials informatics is a growing field in materials science. Materials scientists have begun to use soft computing techniques to discover novel materials. In order to apply these techniques, the descriptors (referred to as features in computer science) of a material must be selected, thereby deciding the resulting performance. As a way of describing a material, the properties of each element in the material are used directly as the features of the input variable. Depending on the number of elements in the material, the dimensionality of the input may differ. Hence, it is not possible to apply the same model to materials with different numbers of elements for tasks such as regression or discrimination. In the present paper, we present a novel method of uniforming the dimensionality of the input that allows regression or discriminative tasks to be performed using soft computing techniques. The main contribution of the proposed method is to provide a solution for uniforming the dimensionality among input vectors of different size. The proposed method is a variant of the denoising autoencoder Vincent et al. (2008) [1] using neural networks and gives a latent representation with uniformed dimensionality of the input. In the experiments of the present study, we consider compounds with ionic conductivity and hydrogen storage materials. The results of the experiments indicate that the regression tasks can be performed using the uniformed latent data learned by the proposed method. Moreover, in the clustering task using these latent data, we observed distance preservation in data space, which is also the case for the denoising autoencoder. This result may enable the proposed method to be used in a broad range of applications.
6. Conclusion
In the present paper, we proposed a uniforming method for the dimensionality of data using a neural network for materials informatics, in which different numbers of elements in compounds are often dealt with, in order to predict the properties of materials or to classify compounds. In addition, we considered the case of material properties for which the underlying mechanisms or theoretical models are poorly understood. Therefore, we introduced a naive representation for the compound. The length of the vector in the naive representation was varied depending on the number of elements. Hence, uniformed dimensionality of the input data was necessary. Unlike conventional methods, such as the multilayer autoencoder, the denoising autoencoder, and kernel PCA, the proposed method can make uniform the dimensionality of data while simultaneously considering the expansion and reduction of the dimensionality. In the proposed method, uniforming of dimensionality is realized by noise injection into the extended part of the input vector during learning for the autoencoder, which is a variant of the denoising autoencoder [1,15,16]. The latent representation in the neural network becomes a uniformed representation of the input data. Experiments on synthetic data, ion conductivity data, and hydrogen storage materials data revealed that the proposed method works well for the linear regression task, as compared to the conventional methods, and exhibits distance preservation and robustness. The results may enable us to apply the proposed method to a broad range of applications. Further study is necessary in order to investigate the statistical properties ofthe uniformed representation of data. Furthermore, in order to improve the performance of the linear regression task, we proposed the linear guided autoencoder, which has a linear guided term in the objective function. In the future, we hope to confirm the effectiveness of the linear guided autoencoder for use in materials informatics.