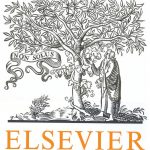
ترجمه مقاله نقش ضروری ارتباطات 6G با چشم انداز صنعت 4.0
- مبلغ: ۸۶,۰۰۰ تومان
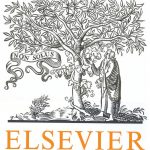
ترجمه مقاله پایداری توسعه شهری، تعدیل ساختار صنعتی و کارایی کاربری زمین
- مبلغ: ۹۱,۰۰۰ تومان
Abstract
This paper proposes a machine learning application to identify mobile phone users suspected of involvement in criminal activities. The application characterizes the behavioral patterns of suspect users versus non-suspect users based on usage metadata such as call duration, call distribution, interaction time preferences and text-to-call ratios while avoiding any access to the content of calls or messages. The application is based on targeted Bayesian network learning method. It generates a graphical network that can be used by domain experts to gain intuitive insights about the key features that can help identify suspect users. The method enables experts to manage the trade-off between model complexity and accuracy using information theory metrics. Unlike other graphical Bayesian classifiers, the proposed application accomplishes the task required of a security company, namely an accurate suspect identification rate (recall) of at least 50% with no more than a 1% false identification rate. The targeted Bayesian network learning method is also used for additional tasks such as anomaly detection, distinction between “relevant” and “irrelevant” anomalies, and for associating anonymous telephone numbers with existing users by matching behavioral patterns.
5 Conclusions
The results obtained by the considered use case show that the TBNL method obtained a 50% recall with a false positive rate of no more than 1%. Note that these results were obtained without accessing the contents of the CDRs; only their metadata were used and analyzed to characterize users’ behavioral patterns. The added value of the TBNL lies in its capability to efficiently manage the trade-off between model complexity and accuracy as well as in its ability to provide an informative graphical interface that allows security domain experts to investigate and find the behavioral patterns that can distinguish suspect from non-suspect users. Regarding this particular use case, the most influential characteristics for the classification task were found to be the durations of the CDRs and their derivatives in various crossovers, such as the average call duration with other suspects and the distribution of calls and text messages throughout the four quarters of the day. We used primary statistical metrics; however, we do not claim that the feature engineering task was optimal, and we leave this discussion for future research. The applied algorithm considers such new features during the BN learning stage while providing an intuitive presentation that subject matter experts can grasp easily.