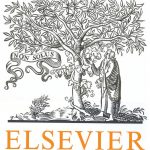
ترجمه مقاله نقش ضروری ارتباطات 6G با چشم انداز صنعت 4.0
- مبلغ: ۸۶,۰۰۰ تومان
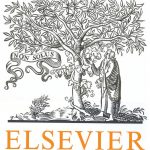
ترجمه مقاله پایداری توسعه شهری، تعدیل ساختار صنعتی و کارایی کاربری زمین
- مبلغ: ۹۱,۰۰۰ تومان
Abstract
We are interested in the reproducibility of face recognition systems. By reproducibility we mean: is the scientific community, and are the researchers from different sides, capable of reproducing the last published results by a big company, that has at its disposal huge computational power and huge proprietary databases? With the constant advancements in GPU computation power and availability of open-source software, the reproducibility of published results should not be a problem. But, if architectures of the systems are private and databases are proprietary, the reproducibility of published results can not be easily attained. To tackle this problem, we focus on training and evaluation of face recognition systems on publicly available data and software. We are also interested in comparing the best Deep Neural Net (DNN) based results with a baseline ”classical” system. This paper exploits the OpenFace open-source system to generate a deep convolutional neural network model using publicly available datasets. We study the impact of the size of the datasets, their quality and compare the performance to a classical face recognition approach. Our focus is to have a fully reproducible model. To this end, we used publicly available datasets (FRGC, MS-celeb-1M, MOBIO, LFW), as well publicly available software (OpenFace) to train our model in order to do face recognition. Our best trained model achieves 97.52% accuracy on the Labelled in the Wild dataset (LFW) dataset which is lower than Google’s best reported results of 99.96% but slightly better than FaceBook’s reported result of 97.35%. We also evaluated our best model on the challenging video dataset MOBIO and report competitive results with the best reported results on this database.
CONCLUSIONS AND PERSPECTIVES
This paper details how to obtain a state-of-the-art face recognition system based on publicly available software and using public datasets. We try to give the most possible details to allow for the reproducibility of the results. When CMU implemented OpenFace, reproduciblity was one of their main goals. Thus we were able to reproduce their results and improve upon them. However, we couldn’t get the same results as Google who used huge proprietary datasets and a proprietary face alignment system. Our OpenFace best DNN model gives good verification results on both evaluation datasets, MOBIO and LFW. From the results that we obtained we can infer that the performance bottleneck lays in the preprocessing, notably the face detection phase. Given enough data, the DNN is unmatched. Nevertheless, in situations where the data are not available classical approaches give better performance. In order to improve our results, we plan to study further the preprocessing and use neural networks for the face detection. We also plan to reduce the mislabelling of the MS-celeb-1M dataset in order to study the effect of the mislabelling on the generalization of the system.