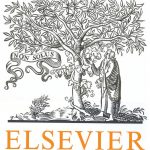
ترجمه مقاله نقش ضروری ارتباطات 6G با چشم انداز صنعت 4.0
- مبلغ: ۸۶,۰۰۰ تومان
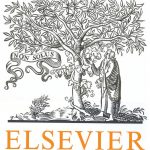
ترجمه مقاله پایداری توسعه شهری، تعدیل ساختار صنعتی و کارایی کاربری زمین
- مبلغ: ۹۱,۰۰۰ تومان
Among radiological imaging data, Chest X-rays (CXRs) are of great use in observing COVID-19 manifestations. For mass screening, using CXRs, a computationally efficient AI-driven tool is the must to detect COVID-19-positive cases from non-COVID ones. For this purpose, we proposed a light-weight Convolutional Neural Network (CNN)-tailored shallow architecture that can automatically detect COVID-19-positive cases using CXRs, with no false negatives. The shallow CNN-tailored architecture was designed with fewer parameters as compared to other deep learning models. The shallow CNN-tailored architecture was validated using 321 COVID-19-positive CXRs. In addition to COVID-19-positive cases, another set of non-COVID-19 5856 cases (publicly available, source: Kaggle) was taken into account, consisting of normal, viral, and bacterial pneumonia cases. In our experimental tests, to avoid possible bias, 5-fold cross-validation was followed, and both balanced and imbalanced datasets were used. The proposed model achieved the highest possible accuracy of 99.69%, sensitivity of 1.0, where AUC was 0.9995. Furthermore, the reported false positive rate was only 0.0015 for 5856 COVID-19-negative cases. Our results stated that the proposed CNN could possibly be used for mass screening. Using the exact same set of CXR collection, the current results were better than other deep learning models and major state-of-the-art works.
Introduction
In December 2019, the novel coronavirus disease (COVID-19) was found in Wuhan Province of China https://www.who.int/docs/default-source/coronaviruse/who -china-joint-mission-on-covid-19-final-report.pdf [1].Unlike the common cold and flu, COVID-19 is much more contagious, and for human’s immune system, it is an absolute unknown. To be more specific, severe acute respiratory syndrome (SARS) and Middle East respiratory syndrome (MERS) are two well-known coronavirus diseases, which have huge mortality rates of 10% and 37%, respectively [2, 3]. As of now, COVID-19 affected more than 6,057,853 people with more than 371,166 death cases across the world (dated, June 01, 2020) [8]. The COVID-19 outbreak spread rate is exponential and is faster than other respiratory-related diseases. Researchers are now limited to small amount of data to predict possible consequences using AI-driven tools.
Conclusion and Future Works
On the whole, in this paper, a light-weight CNN-tailored shallow architecture was proposed to detect COVID-19- positive cases using CXRs against non-COVID-19 ones. The experiments were performed on dataset collection of COVID19-positive, pneumonia-positive, and healthy CXRs. To validate its robustness, 5-fold cross-validation protocol was used on both balanced and imbalanced dataset scenarios, where 321 COVID-19-positive cases were used. We have compared the proposed shallow CNN-tailored architecture by taking (a) popular DL tools, such as MobileNet, InceptionV3, and ResNet50; and (b) state-of-the-art works for COVID-19 detection using CXRs, into account. The proposed model outperformed all and is computationally efficient as it requires less number of parameters. As the proposed shallow CNNtailored architecture has no false negatives, it could be used to screen COVID-19-positive cases in chest X-rays.
In future, the network architecture will be explored, aiming to minimize false positive cases. We also plan to augment data that helps avoid an issue we currently face, i.e., lack of sufficient data.
در میان دادههای تصویربرداری رادیولوژی، عکسبرداری اشعه ایکس قفسه سینه (CXR) در مشاهده بروز کووید 19 بسیار استفاده میشود. برای غربالگری انبوه، استفاده از CXR، یک ابزار مبتنی بر هوش مصنوعی محاسباتی کارآمد، برای تشخیص موارد مثبت کووید 19 از موارد غیر کووید ضروری است. به این منظور، یک شبکه عصبی کانولوشن سبک وزن (CNN) با معماری کم عمق متناسب ارائه داده ایم که با استفاده از CXR موارد مثبت کووید 19 را بدون منفی کاذب بطور خودکار تشخیص میدهد. معماری کم عمق CNN در مقایسه با سایر مدلهای یادگیری عمیق با پارامترهای کمتری طراحی شده است. معماری متناسب با CNN کم عمق با استفاده از CXRهای 321 مورد مثبت کووید تأیید شد. علاوه بر موارد مثبت کووید 19، مجموعه دیگری از 5856 مورد غیرکووید 19 (در دسترس عموم، منبع: کاگل) در نظر گرفته شد که شامل موارد ذات الریه عادی، ویروسی و باکتریایی است. در آزمونهای آزمایشی ما، برای جلوگیری از جهتگیری احتمالی، اعتبارسنجی 5 برابر دنبال شد و از هر دو مجموعه داده متعادل و نامتعادل استفاده شد. مدل پیشنهادی بالاترین دقت ممکن 99.69٪، را با حساسیت 1.0 به دست آورد، جایی که AUC، 9995/0 بود. بعلاوه، میزان مثبت کاذب گزارش شده برای 5856 مورد منفی کووید19 فقط 0015/0 بود. نتایج ما اظهار داشت که CNN پیشنهادی میتواند برای غربالگری انبوه استفاده شود. با استفاده از مجموعه دقیقاً مشابه مجموعه CXR، نتایج فعلی بهتر از سایر مدلهای یادگیری عمیق و کارهای پیشرفته اصلی بود.
مقدمه
در دسامبر سال 2019، بیماری جدید ویروس کرونا (COVID - 19) در استان ووهان چین یافت شد https://www.who.int/docs/default-source/coronaviruse/who - china-joint-mission-on-covid-19-final-report.pdf [1]. برخلاف سرماخوردگی و آنفولانزا، کووید19 بسیار مسری تر است و برای سیستم ایمنی بدن انسان کاملاً ناشناخته است. به بیان دقیقتر، سندرم تنفسی بسیار حاد (SARS) و سندرم تنفسی خاورمیانه (MERS) دو بیماری شناخته شده ویروس کرونا هستند که به ترتیب 10 و 37 درصد مرگ و میر زیادی دارند [2، 3]. هم اکنون، کووید 19 بیش از 6،057،853 نفر را مبتلا کرده است که بیش از 371،166 مورد مرگ در سراسر جهان دارند (تاریخ، 01 ژوئن، 2020) [8]. نرخ شیوع کووید19 نمایی است و سریعتر از سایر بیماریهای مربوط به تنفس است. امروزه محققان برای پیش بینی عواقب احتمالی با استفاده از ابزارهایی که توسط هوش مصنوعی هدایت میشوند، به دادههای کمی محدود شدهاند.
نتیجهگیری و کارهای آینده
به طور کلی، در این مقاله، یک معماری کم عمق متناسب با CNN کم وزن را برای تشخیص موارد مثبت کووید 19 با استفاده از CXRها در برابر موارد غیر کووید 19 پیشنهاد شده است. این آزمایشات بر روی مجموعه داده CXRهای کویید 19 مثبت، ذاتالریه - مثبت و سالم انجام شد. برای تأیید دقت، از پروتکل اعتبار سنجی 5 برابر در هر دو سناریوی مجموعه داده متعادل و نامتعادل استفاده شد که در آن 321 مورد مثبت کووید 19 استفاده شده است. با استفاده از (الف) ابزارهای محبوب DL، مانند MobileNet، InceptionV3 و ResNet50، معماری متناسب CNN پیشنهادی را مقایسه کرده ایم. و (ب) کارهای پیشرفته تشخیص COVID - 19 با استفاده از CXRها، در نظر گرفته شود. مدل پیشنهادی بهتر از همه بوده و از نظر محاسباتی کارآمد است زیرا به تعداد پارامترهای کمتری نیاز دارد. چون معماری CNN کم عمق پیشنهادی منفی کاذب ندارد، میتوان از آن برای غربالگری موارد مثبت کووید 19 در اشعه X قفسه سینه استفاده کرد. در آینده،
معماری شبکه با هدف به حداقل رساندن موارد مثبت کاذب، بررسی میشود. همچنین قصد داریم دادهها را اضافه کنیم که به ما کمک میکند تا از مشکلی که در حال حاضر با آن روبرو هستیم، یعنی کمبود داده کافی، جلوگیری کنیم.