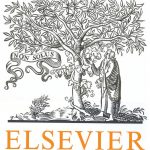
ترجمه مقاله نقش ضروری ارتباطات 6G با چشم انداز صنعت 4.0
- مبلغ: ۸۶,۰۰۰ تومان
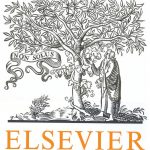
ترجمه مقاله پایداری توسعه شهری، تعدیل ساختار صنعتی و کارایی کاربری زمین
- مبلغ: ۹۱,۰۰۰ تومان
Abstract
In order to detect the cerebral microbleed (CMB) voxels within brain, we used susceptibility weighted imaging to scan the subjects. Then, we used undersampling to solve the accuracy paradox caused from the imbalanced data between CMB voxels and non-CMB voxels. we developed a seven-layer deep neural network (DNN), which includes one input layer, four sparse autoencoder layers, one softmax layer, and one output layer. Our simulation showed this method achieved a sensitivity of 95.13%, a specificity of 93.33%, and an accuracy of 94.23%. The result is better than three state-of-the-art approaches.
Conclusions
In this study, our team proposed a new 7-layer SAE based deep neural network for cerebral microbleed detection. The results showed that this method is promising and gives better results than three state-of-the-art methods: MRST + RF [52], LReLU [9], and 4-layer DNN [24]. In the future, we shall enroll more subjects to increase the reliability and robustness of our method. Besides, we shall test other advanced classifiers, such as linear regression classifier, extreme learning machine, etc. Acknowledgements This paper was supported by NSFC (61602250), Leading Initiative for Excellent Young Researcher (LEADER) of Ministry of Education, Culture, Sports, Science and Technology-Japan (16809746), Natural Science Foundation of Jiangsu Province (BK20150983), Program of Natural Science Research of Jiangsu Higher Education Institutions (16KJB520025), Open Research Fund of Hunan Provincial Key Laboratory of Network Investigational Technology (2016WLZC013), Open Fund of Fujian Provincial Key Laboratory of Data Intensive Computing (BD201607), Open fund for Jiangsu Key Laboratory of Advanced Manufacturing Technology (HGAMTL1601), Open fund of Key Laboratory of Guangxi High Schools Complex System and Computational Intelligence (2016CSCI01).