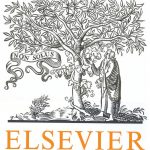
ترجمه مقاله نقش ضروری ارتباطات 6G با چشم انداز صنعت 4.0
- مبلغ: ۸۶,۰۰۰ تومان
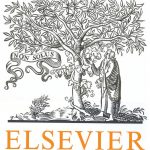
ترجمه مقاله پایداری توسعه شهری، تعدیل ساختار صنعتی و کارایی کاربری زمین
- مبلغ: ۹۱,۰۰۰ تومان
Abstract
Trustworthiness is a comprehensive quality metric which is used to assess the quality of the services in service-oriented environments. However, trust prediction of cloud services based on the multi-faceted Quality of Service (QoS) attributes is a challenging task due to the complicated and non-linear relationships between the QoS values and the corresponding trust result. Recent research works reveal the significance of Artificial Neural Network (ANN) and its variants in providing a reasonable degree of success in trust prediction problems. However, the challenges with respect to weight assignment, training time and kernel functions make ANN and its variants under continuous advancements. Hence, this work presents a novel multi-level Hypergraph Coarsening based Robust Heteroscedastic Probabilistic Neural Network (HC-RHRPNN) to predict trustworthiness of cloud services to build high-quality service applications. HC-RHRPNN employs hypergraph coarsening to identify the informative samples, which were then used to train HRPNN to improve its prediction accuracy and minimize the runtime. The performance of HC-RHRPNN was evaluated using Quality of Web Service (QWS) dataset, a public QoS dataset in terms of classifier accuracy, precision, recall, and F-Score.
Conclusions
Trustworthiness plays a significant role in determining the quality of the candidate services for the design of efficient and resilient service-based systems. Recent research trends in cloud service selection signifies the impact and popularity of trust based cloud service selection models due to fact that trustworthiness of a service is well-reflected by several functional and non-functional QoS attributes. However, the dynamic nature of service-oriented environments in terms of variable QoS values and emergence of new cloud services affects the performance of trust-based cloud service selection models. Trust prediction, a classification problem can be modeled as a suitable solution for the trust based cloud service selection problem through predicting the trustworthiness of the cloud services based on their relevant historical QoS information. Therefore, several researchers focused towards the development of numerous trust and QoS prediction models based on machine learning and statistical techniques. Artificial Neural Networks (ANN) and its variants have proven their significance in solving service selection problems with high prediction accuracy. However, several challenges related to weights, training time, and kernel functions makes the development of an efficient and stable neural network architecture, an open research challenge. Hence, this work presents a multi-level Hypergraph Coarsening based Robust Heteroscedastic Probabilistic Neural Network (HC-RHRPNN) to predict the trustworthiness of services in cloud environments. HC-RHRPNN uses hypergraph coarsening for the identification of informative samples, which were then used to train HRPNN to achieve high prediction accuracy and to minimize the runtime. The predominance of HCRHRPNN was proved with a set of extensive experiments on Quality of Web Service (QWS), a public QoS dataset in terms of classifier accuracy, precision, recall, and F-Score. Further, HC-RHRPNN was found to be adaptive, efficient, scalable, and applicable for classification and prediction problems in intrusion detection systems, energy prediction, stock market analysis, medical diagnosis, and metadata quality analysis.