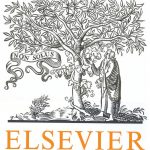
ترجمه مقاله نقش ضروری ارتباطات 6G با چشم انداز صنعت 4.0
- مبلغ: ۸۶,۰۰۰ تومان
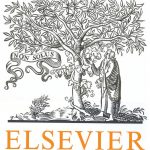
ترجمه مقاله پایداری توسعه شهری، تعدیل ساختار صنعتی و کارایی کاربری زمین
- مبلغ: ۹۱,۰۰۰ تومان
Abstract
Neural networks are used in many applications such as image recognition, classification, control and system identification. However, the parameters of the identified system are embedded within the neural network architecture and are not identified explicitly. In this paper, a mathematical relationship between the network weights and the transfer function parameters is derived. Furthermore, an easy-to-follow algorithm that can estimate the transfer function models for multi-layer feedforward neural networks is proposed. These estimated models provide an insight into the system dynamics, where information such as time response, frequency response, and pole/zero locations can be calculated and analyzed. In order to validate the suitability and accuracy of the proposed algorithm, four different simulation examples are provided and analyzed for three-layer neural network models.
5. Conclusion
Researchers have used neural network models for system identification applications with good success. However, these network models hide the parametric information of the system within their structures. In this paper, a clear mathematical relationship between the network weights and the system parameters was established in order to calculate estimated transfer functions. Parametric identification can provide valuable information and insight about the system’s behavior such as the pole/zero locations and bode plots. In order to make the presented work valuable for other researchers, an easy-to-follow algorithm, NN2TF, was derived, analyzed, and validated. This algorithm has the capability of transforming the weights of a multi-layer neural network into an accurate transfer functionand canbeused by researchers to capture important information about their identified systems. Simulation runs for four different models were used to validate the algorithm’s results. First, two linear models were used to validate the accuracy ofthe estimated transfer functions (using NN2TF) as their time and frequency responses were compared to the original and ANN models. Then, a nonlinear model was generated to test the capability of the proposed algorithm to generate accurate transfer functions. All results showed that the estimated transfer function was able to mimic the behavior ofthe original models with minimum error. Finally, a third nonlinear model was used to show that the algorithm was able to adjust its converged parameters in order to produce stable models.