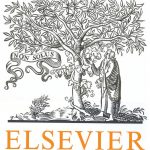
ترجمه مقاله نقش ضروری ارتباطات 6G با چشم انداز صنعت 4.0
- مبلغ: ۸۶,۰۰۰ تومان
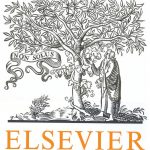
ترجمه مقاله پایداری توسعه شهری، تعدیل ساختار صنعتی و کارایی کاربری زمین
- مبلغ: ۹۱,۰۰۰ تومان
Abstract
Selection of optimal features is an important area of research in medical data mining systems. In this paper we introduce an efficient four-stage procedure – feature extraction, feature subset selection, feature ranking and classification, called as Multi-Filtration Feature Selection (MFFS), for an investigation on the improvement of detection accuracy and optimal feature subset selection. The proposed method adjusts a parameter named ‘‘variance coverage’’ and builds the model with the value at which maximum classification accuracy is obtained. This facilitates the selection of a compact set of superior features, remarkably at a very low cost. An extensive experimental comparison of the proposed method and other methods using four different classifiers (Naı¨ve Bayes (NB), Support Vector Machine (SVM), multi layer perceptron (MLP) and J48 decision tree) and 22 different medical data sets confirm that the proposed MFFS strategy yields promising results on feature selection and classification accuracy for medical data mining field of research.
5. Conclusion
In this paper, we have proposed an efficient Multi Filtration Feature Selection (MFFS) method applicable to medical data mining. Empirical study on 6 synthetic medical datasets suggests that MFFS gives better over-all performance than the existing counterparts in terms of all three evaluation criteria, i.e., number of selected features, classification accuracy, and computational time. The comparison to other methods in the literature also suggests MFFS has competitive performance. MFFS is capable of eliminating irrelevant and redundant features based on both feature subset selection and ranking models effectively, thus providing a small set of reliable features for the physicians to prescribe further medications. For simplicity, several key points are collected as follows. (1) It seems that the classification performance is necessarily proportional to the removal of redundant features, heavily dependent on the inclusion of relevant features and the ‘‘Accuracy’’ metric is observed maximum with minimum number of features. (2) The proposed MFFS algorithm operates invariably well on any type of classifier model. This shows the generalization ability and applicability of the proposed system. (3) Our training and test database collects the popular and benchmark medical datasets. However, the proposed method can be tested and applied on real-world dataset too. (4) The best accuracy rate achieved by our proposed system is superior to the existing schemes. To make our system more practical, future work could include the following. (a) Fitting the proposed system to classify any other realworld dataset. (b) Applying the proposed method for a multi-label dataset, where a record may belong to many classes simultaneously.