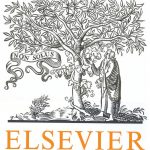
ترجمه مقاله نقش ضروری ارتباطات 6G با چشم انداز صنعت 4.0
- مبلغ: ۸۶,۰۰۰ تومان
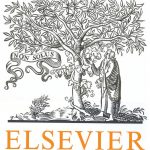
ترجمه مقاله پایداری توسعه شهری، تعدیل ساختار صنعتی و کارایی کاربری زمین
- مبلغ: ۹۱,۰۰۰ تومان
Abstract
This paper addresses the task of identification of nonlinear dynamic systems from measured data. The discrete-time variant of this task is commonly reformulated as a regression problem. As tree ensembles have proven to be a successful predictive modeling approach, we investigate the use of tree ensembles for solving the regression problem. While different variants of tree ensembles have been proposed and used, they are mostly limited to using regression trees as base models. We introduce ensembles of fuzzified model trees with split attribute randomization and evaluate them for nonlinear dynamic system identification. Models of dynamic systems which are built for control purposes are usually evaluated by a more stringent evaluation procedure using the output, i.e., simulation error. Taking this into account, we perform ensemble pruning to optimize the output error of the tree ensemble models. The proposed Model-Tree Ensemble method is empirically evaluated by using input–output data disturbed by noise. It is compared to representative state-of-the-art approaches, on one synthetic dataset with artificially introduced noise and one real-world noisy data set. The evaluation shows that the method is suitable for modeling dynamic systems and produces models with comparable output error performance to the other approaches. Also, the method is resilient to noise, as its performance does not deteriorate even when up to 20% of noise is added.
4. Conclusions, discussion, and further work
4.1. Summary We address the task of discrete-time modeling of nonlinear dynamic systems using measured data, which is typically converted into a regression problem. We investigate the use of tree ensembles for regression, a very successful predictive modeling approach, for this task. We consider existing tree ensemble approaches to regression, such as bagging of model trees, and propose the use of a novel approach of learning model tree ensembles tailored to the task of modeling dynamic systems. The latter learns random forests of fuzzified model trees and performs ensemble selection based on the output error measure. We evaluate the performance of the tree ensemble methods and three state-of-the-art methods for system identification typically used in control engineering. We consider the predictive performance of the learned models and in particular their resilience to noise. For this purpose, we use one synthetic task without noise, one synthetic task with different levels of artificially introduced noise, and one real task of modeling nonlinear dynamic systems, all coming from the area of control engineering.