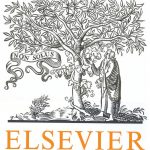
ترجمه مقاله نقش ضروری ارتباطات 6G با چشم انداز صنعت 4.0
- مبلغ: ۸۶,۰۰۰ تومان
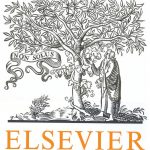
ترجمه مقاله پایداری توسعه شهری، تعدیل ساختار صنعتی و کارایی کاربری زمین
- مبلغ: ۹۱,۰۰۰ تومان
ABSTRACT
Municipal solid waste management represents an increasingly significant environmental, fiscal, and social challenge for cities. Understanding patterns of municipal waste generation behavior at the household and building scales is a critical component of efficient collection routing and the design of incentives to encourage recycling and composting. However, high spatial resolution estimates of building refuse and recycling have been constrained by the lack of granular data for individual properties. This paper presents a new analytical approach, which combines machine learning and small area estimation techniques, to predict weekly and daily waste generation at the building scale. Using daily collection data from 609 New York City Department of Sanitation (DSNY) sub-sections over ten years, together with detailed data on individual building attributes, neighborhood socioeconomic characteristics, weather, and selected route-level collection data, we apply gradient boosting regression trees and neural network models to estimate daily and weekly refuse and recycling tonnages for each of the more than 750,000 residential properties in the City. Following cross-validation and a two-stage spatial validation, our results indicate that our method is capable of predicting building-level waste generation with a high degree of accuracy. Our methodology has the potential to support collection truck route optimization based on expected building-level waste generation rates, and to facilitate new equitable solid waste management policies to shift behavior and divert waste from landfills based on benchmarking and peer performance comparisons.
5. Discussion, limitations, and conclusion
The aim of this research is to develop a predictive model for waste generation at the building-level in a dense urban environment, using New York City as a case study. We combine a socio-spatial model of waste generation per capita per week with estimates of the occupant population for each of the more than 750,000 residential buildings in the City. Our best-performing predictive model (GBRT) is able to predict total weekly waste generation for DSNY sub-sections with an outof-sample R-squared value of 0.87. Subsequent models built predicting refuse, MGP, and paper recycling, respectively, also perform well. We find that the variables with the highest feature importance are weather (temperature, precipitation, wind speed, and snow event), residential building type and density, and demographic variables. Weather-related features capture temporal (i.e. seasonal) variations in the data, in addition to capturing weekly anomalous weather activity. Our building prediction model demonstrates high levels of accuracy following two validation processes. In the two collection truck validation cases, the model resulted in 99.8% and 93.9% prediction accuracy, respectively.
Certain data limitations constrain the predictive power of our model, although iterative improvements are expected as additional validation data are acquired. In order to reflect the spatial heterogeneity in waste generation behavior and the propensity of a unit in a building to be occupied, additional features should be considered. Our model could be improved with accurate information on building occupancies at high temporal resolution, particularly accounting for weekly fluctuations in residential population. In addition, specific information on the waste set-out (pick-up/drop-off) point for each building would be useful to match buildings to their true truck route narratives. We are currently working with DSNY to collect these data across selected routes.