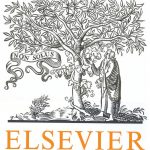
ترجمه مقاله نقش ضروری ارتباطات 6G با چشم انداز صنعت 4.0
- مبلغ: ۸۶,۰۰۰ تومان
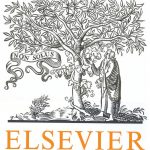
ترجمه مقاله پایداری توسعه شهری، تعدیل ساختار صنعتی و کارایی کاربری زمین
- مبلغ: ۹۱,۰۰۰ تومان
Abstract
This paper proposes a new conditional kernel CCA (canonical correlation analysis) algorithm and exploits statistical consistency of it via modified Tikhonov regularization scheme, which is a continuous study of [11]. A new measure which characterizes consistency of learning ability is discussed based on the notion of distance between feature subspaces. The consistency analysis is conducted under the assumptions of normalized cross-covariance operators, which is mild and can be constructed by means of mean square contingency. Meantime, the relationship between this new measure and previous consistency scheme is investigated. Furthermore, we study conditional kernel CCA in a more general scenario by means of the trace operator.
5. Conclusions
In this paper, we introduce a new conditional kernel CCA algorithm motivated by the conditional dependence measure presented in [11] and the discussion about kernel CCA in [10]. The algorithm and theoretical analysis for conditional CCA are elegantly conducted under mild conditions on VY X, VY Z and VZX. We demonstrate that these conditions are closely related with mean square contingency as indicated in Section 2. Meantime, the convergence rates of empirical NCCCO to NCCCO are conducted under the above conditions in the sense of Hilbert–Schmidt norm, which is the extension of Theorem 5 in [11]. Moreover, the multiple extension of conditional kernel CCA has also been addressed in Section 3, which can be viewed as a generalization of Theorem 3.1 in [5]. There are some practical problems that remain to be addressed for conditional kernel CCA. One is how to choose the regularization constant εm in practice. The final convergence rates of our algorithm are “dragged slow” due to the sufficient condition of εm. That is εm = m−α, 0 <α< 1 3 . This problem should be studied more in our future work. Moreover, how to find simpler conditions than AA and improve the convergence rates of conditional kernel CCA will be investigated in the future. Another important unsolved problem is the choice of kernel. Kernel method is efficient for detecting nonlinear relations between variables. Successful applications of kernel-based algorithms are widespread in the community of learning theory. Thus, in order to improve the learning rates of conditional kernel CCA, how to choose an optimal combination of kernels is crucial in the literature of CCA related problems. A combination of Gaussian kernel and polynomial kernel was studied in [26] for kernel CCA problem, which shows good performance in the community of kernel learning. But the theoretical analysis of it is still not clear and this will be investigated in the future work.