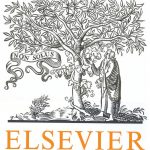
ترجمه مقاله نقش ضروری ارتباطات 6G با چشم انداز صنعت 4.0
- مبلغ: ۸۶,۰۰۰ تومان
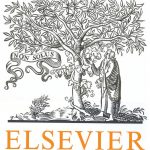
ترجمه مقاله پایداری توسعه شهری، تعدیل ساختار صنعتی و کارایی کاربری زمین
- مبلغ: ۹۱,۰۰۰ تومان
Abstract
Incremental learning has been developed for supervised classification, where knowledge is accumulated incrementally and represented in the learning process. However, labeling sufficient samples in each data chunk is of high cost, and incremental technologies are seldom discussed in the semi-supervised paradigm. In this paper we advance an Incremental Semi-Supervised classification approach via Self-Representative Selection (IS3RS) for data streams classification, by exploring both the labeled and unlabeled dynamic samples. An incremental self-representative data selection strategy is proposed to find the most representative exemplars from the sequential data chunk. These exemplars are incrementally labeled to expand the training set, and accumulate knowledge over time to benefit future prediction. Extensive experimental evaluations on some benchmarks have demonstrated the effectiveness of the proposed framework.
4. Conclusion
In this paper, we proposed a new incremental semi-supervised learning framework via representation learning for stream data classification. The key idea of this new algorithm is to improve the classification performance based on the information incrementally learned from the testing data. Representative learning is used to obtain informative exemplars of the stream data, and co-training technique is used to label the exemplars. We investigate the effectiveness of the proposed algorithm on some benchmark datasets, and compare it with some state-of-the-art results on incremental learning. The results show that our method can find informative exemplars to enlarge the training set and gradually find new classes. Moreover, our method can achieve higher classification results than its counterparts. The proposed algorithm has potential business applications in stock forecasting and other data mining tasks. So it can be embedded into a business forecasting software to deal with large scale data streams or “big” dataset. Future work will be taken on an extension of our method to a distributed version and a realization on a parallel computing platform.