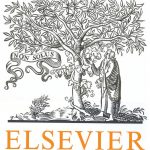
ترجمه مقاله نقش ضروری ارتباطات 6G با چشم انداز صنعت 4.0
- مبلغ: ۸۶,۰۰۰ تومان
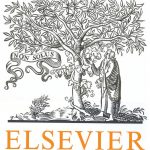
ترجمه مقاله پایداری توسعه شهری، تعدیل ساختار صنعتی و کارایی کاربری زمین
- مبلغ: ۹۱,۰۰۰ تومان
Abstract
The focus of this study is to use Monte Carlo method in fuzzy linear regression. The purpose of the study is to figure out the appropriate error measures for the estimation of fuzzy linear regression model parameters with Monte Carlo method. Since model parameters are estimated without any mathematical programming or heavy fuzzy arithmetic operations in fuzzy linear regression with Monte Carlo method. In the literature, only two error measures (E1 and E2) are available for the estimation of fuzzy linear regression model parameters. Additionally, accuracy of available error measures under the Monte Carlo procedure has not been evaluated. In this article, mean square error, mean percentage error, mean absolute percentage error, and symmetric mean absolute percentage error are proposed for the estimation of fuzzy linear regression model parameters with Monte Carlo method. Moreover, estimation accuracies of existing and proposed error measures are explored. Error measures are compared to each other in terms of estimation accuracy; hence, this study demonstrates that the best error measures to estimate fuzzy linear regression model parameters with Monte Carlo method are proved to be E1, E2, and the mean square error. One the other hand, the worst one can be given as the mean percentage error. These results would be useful to enrich the studies that have already focused on fuzzy linear regression models.
6. Conclusions
6.1. General findings In this study, we use different error measures to find parameter estimates of fuzzy linear regression models with MC method. Regression models are applied to two cases. In the first one, input data is crisp and output data is fuzzy (Case-II) and in the second case, input and output data are both fuzzy (Case-III). We utilize a more general definition of absolute value of a triangular shaped fuzzy number given by AbuAraqub et al. [22] in our MC method. A simulation study is conducted to compare estimation performances of the selected error measures. It is demonstrated that only two error measures (E1 and E2) are not sufficient to estimate parameters of fuzzy linear regression models. Additionally, parameter estimates that make each error measure minimum are calculated. The differences between the true and estimated values of the parameters are evaluated by using MAEc and MSEc. These error measures are preferred because of the fact that MSEc at least has two advantages over other distance measures: First, itis analytically tractable and second, it has an interpretation. Besides, MAEc is a reasonable alternative of MSEc. Also, the parameters are estimated by considering five different intervals that possibly include their true values. Each interval (Ii, i = 0, 1, 2, 3, 4) is arbitrarily determined according to its length and status of involving any negative number. The results of simulation study are generalized as follows. Considering Case-II, minimum error values are observed in E1, E2, and MSEe. On the other hand, maximum errors are often produced by MPEe regarding both MAEc and MSEc. Considering Case-III, minimum error values are obtained by E1, E2, and MSEe. However, maximum errors are often observed by MPEe in terms of both MAEc and MSEc. As a result, itis possible to conclude that best error measures to estimate fuzzy/crisp parameters of fuzzy linear regression models are not only E1 and E2 but also MSEe. Furthermore,the worst error measure is proved to be MPEe for estimating the parameters of fuzzy linear regression models.