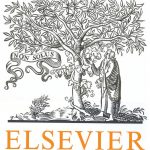
ترجمه مقاله نقش ضروری ارتباطات 6G با چشم انداز صنعت 4.0
- مبلغ: ۸۶,۰۰۰ تومان
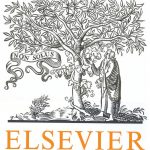
ترجمه مقاله پایداری توسعه شهری، تعدیل ساختار صنعتی و کارایی کاربری زمین
- مبلغ: ۹۱,۰۰۰ تومان
Abstract
Learning the conditional probability table (CPT) parameters of Bayesian networks (BNs) is a key challenge in real-world decision support applications, especially when there are limited data available. A conventional way to address this challenge is to introduce domain knowledge/expert judgments that are encoded as qualitative parameter constraints. In this paper we focus on a class of constraints which is naturally encoded in the edges of BNs with monotonic influences. Experimental results indicate that such monotonic influence constraints are widespread in practical BNs (all BNs used in the study contain such monotonic influences). To exploit expert knowledge about such constraints we have developed an improved constrained optimization algorithm, which achieves good parameter learning performance using these constraints, especially when data are limited. Specifically, this algorithm outperforms the previous state-of-the-art and is also robust to errors in labelling the monotonic influences. The method is applied to a real world medical decision support BN where we had access to expert-provided constraints and real hospital data. The results suggest that incorporating expert judgments about monotonic influence constraints can lead to more accurate BNs for decision support and risk analysis.
7. Conclusions and future work
When data are limited, purely data driven BN learning is inaccurate. In this paper our focus is on those scenarios in which we have a BN whose structure is expert-defined, but whose parameters we seek to learn from a combination of scarce data and expert judgments. By incorporating monotonic influence constraints discussed in this paper parameter learning performance is significantly improved. The broad goal of this paper was to understand the monotonic influence constraints in a range of BNs, and to determine the extent to which knowledge of such constraints improved learning performance. We analysed such properties in each edge of every readable BN in the publicly available BN repository. Surprisingly, monotonic influences were widespread in all the BNs (typically over 40% of all edges in most of the 12 BNs used in the study). We described an improved parameter learning algorithm (COFP) that incorporates constraints generated from these monotonic influences, and compared its performance to MLE, MAP and the previous state-of-the-art algorithm CO using a range of different sample size settings.