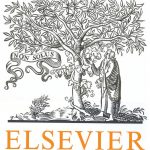
ترجمه مقاله نقش ضروری ارتباطات 6G با چشم انداز صنعت 4.0
- مبلغ: ۸۶,۰۰۰ تومان
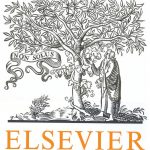
ترجمه مقاله پایداری توسعه شهری، تعدیل ساختار صنعتی و کارایی کاربری زمین
- مبلغ: ۹۱,۰۰۰ تومان
Abstract
Data envelopment analysis (DEA) is a self-evaluation method which assesses the relative efficiency of a particular decision making unit (DMU) within a group of DMUs. It has been widely applied in real-world scenarios, and traditional DEA models with a limited number of variables and linear constraints can be computed easily. However, DEA using big data involves huge numbers of DMUs, which may increase the computational load to beyond what is practical with traditional DEA methods. In this paper, we propose novel algorithms to accelerate the computation process in the big data environment. Specifically, we firstly use an algorithm to divide the large scale DMUs into small scale and identify all strongly efficient DMUs. If the strongly efficient DMU set is not too large, we can use the efficient DMUs as a sample set to evaluate the efficiency of inefficient DMUs. Otherwise, we can identify two reference points as the sample in the situation of just one input and one output. Furthermore, a variant of the algorithm is presented to handle cases with multiple inputs or multiple outputs, in which some of the strongly efficient DMUs are reselected as a reduced-size sample set to precisely measure the efficiency of inefficient DMUs. Last, we test the proposed methods on simulated data in various scenarios.
Conclusions
DEA is an effective tool which has been widely applied in evaluating the efficiency of DMUs. Using a self-evaluation mode, it measures relative efficiency of a DMU by comparing it against a peer group. The traditional DEA models can be solved by using standard linear programming techniques, thus, are theoretically considered computationally easy. However, with big data envelopment, a great number of DMUs need to be evaluated. The large DMU set significantly increases the computation time in a nonlinear fashion and yields challenges for many applications. To overcome these disadvantages of DEA in the big data environment, in this paper we present novel algorithms to accelerate the computation process. Firstly, Algorithm 1 is proposed to divide the large DMU set into groups with a small number of DMUs to reduce the computational burden and identify all strongly efficient DMUs quickly. Using only the strongly efficient DMUs as the sample for evaluating inefficient DMU efficiency can accelerate the computation and thereby save time. Furthermore, if the strongly efficient DMUs also form a large set, further saving of time can be obtained with the proposed algorithms. Two situations are considered: one-input-one-output and multiple-input-multiple-output. In the one-input-one-output situation, we quickly identify two reference points to evaluate the efficiency values for inefficient DMUs. In the situation of multiple inputs or multiple outputs, we use Algorithm 2 to reselect some strongly efficient DMUs as the sample for inefficient DMUs. Last, the proposed methods were tested for effectiveness using simulated data in various scenarios. Some further research directions can be drawn from our study. Firstly, our proposed method just considers the traditional input-oriented and output-oriented BCC models, and hence it can extend to other DEA models, e.g. SMB model. Secondly, the thoughts of divide the huge DMUs into groups in the proposed algorithms can also be used to solve other problems (e.g., resource allocation, environmental problems, etc.) in the context of big data. Finally, DEA can also be extended as a data mining tool to identify and excavate more meaningful information in the big data environment.