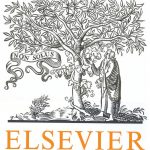
ترجمه مقاله نقش ضروری ارتباطات 6G با چشم انداز صنعت 4.0
- مبلغ: ۸۶,۰۰۰ تومان
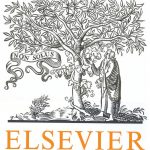
ترجمه مقاله پایداری توسعه شهری، تعدیل ساختار صنعتی و کارایی کاربری زمین
- مبلغ: ۹۱,۰۰۰ تومان
Alzheimer’s disease (AD) is a progressive, neurodegenerative brain disorder that attacks neurotransmitters, brain cells, and nerves, affecting brain functions, memory, and behaviors and then finally causing dementia on elderly people. Despite its significance, there is currently no cure for it. However, there are medicines available on prescription that can help delay the progress of the condition. Thus, early diagnosis of AD is essential for patient care and relevant researches. Major challenges in proper diagnosis of AD using existing classification schemes are the availability of a smaller number of training samples and the larger number of possible feature representations. In this paper, we present and compare AD diagnosis approaches using structural magnetic resonance (sMR) images to discriminate AD, mild cognitive impairment (MCI), and healthy control (HC) subjects using a support vector machine (SVM), an import vector machine (IVM), and a regularized extreme learning machine (RELM). The greedy scorebased feature selection technique is employed to select important feature vectors. In addition, a kernel-based discriminative approach is adopted to deal with complex data distributions. We compare the performance of these classifiers for volumetric sMR image data from Alzheimer’s disease neuroimaging initiative (ADNI) datasets. Experiments on the ADNI datasets showed that RELM with the feature selection approach can significantly improve classification accuracy of AD from MCI and HC subjects.
Conclusions and Future Work
The early diagnosis of AD and MCI is essential for patient care and research, and it is widely accepted that preventive measures plays an important role to delay or alleviate the progression of AD. For the classification task of different stages of AD progression, the smaller number of training samples and the larger number of feature representations are the major challenges. In this study, we investigated SVM, IVM, and RELM for the classification problem. In IVM, only the subsets of the input vectors of KLR are selected by minimizing the regularized cost function to reduce computation time. RELM is an effective solution for SLFNs implemented without iteratively tuning the artificial hidden nodes and adopts reliability-based classification where ELM is adopted if the test data is correctly classified, and sparse representation is selected for the other cases. Experiments on the ADNI dataset showed that RELM-based classifier could significantly improve accuracy in both binary and multiclass classification tasks. In addition, we could observe that adoption of the PCA-based feature selection could improve the accuracy slightly. While this study is focusing on the stage diagnosis of AD progression using sMRI alone, further study is still being carried out to improve the accuracy by elaborating the classifiers, possibly using a model ensemble approach, and feature selection. Also, the studies of adding more modalities such as fMRI and PET in combination with sMRI are also one of our future researches.