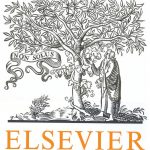
ترجمه مقاله نقش ضروری ارتباطات 6G با چشم انداز صنعت 4.0
- مبلغ: ۸۶,۰۰۰ تومان
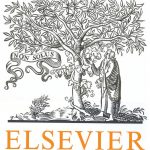
ترجمه مقاله پایداری توسعه شهری، تعدیل ساختار صنعتی و کارایی کاربری زمین
- مبلغ: ۹۱,۰۰۰ تومان
Abstract
In this paper, we define the concept of incomplete hesitant fuzzy preference relations to deal with the cases where the decision makers express their judgments by using hesitant fuzzy preference relations with incomplete information, and investigate the consistency of the incomplete hesitant fuzzy preference relations and obtain the reliable priority weights. We first establish a goal programming model for deriving the priority weights from incomplete hesitant fuzzy preference relations based the α -normalization. Then, we give the definition of multiplicative consistent incomplete hesitant fuzzy preference relations based on the β-normalization, and develop a method for complementing the acceptable incomplete hesitant fuzzy preference relations by using the multiplicative consistency property. Furthermore, utilizing a convex combination method, a new algorithm for obtaining the priority weights from complete or incomplete hesitant fuzzy preference relations is presented on the basis of the β-normalization. Finally, several numerical examples are provided to illustrate the validity and practicality of the proposed methods.
5. Conclusions
In the process of group decision making, the decision makers may uses incomplete hesitant fuzzy preference relations to express their preference information due to the complexity, uncertainty, and hesitancy involved in real decision problems. In order to correctly operation on incomplete HFPRs, we have two principles to normalize them, i.e., the ˛-normalization and the ˇ-normalization. The ˛-normalization reduces a HFPR to a crisp FPR by removing some preference values of some elements of the HFPR, while the ˇ-normalization adds some preference values to some elements which have less lengths than the other ones until all the elements of the HFPR have the same length. Based on the ˛-normalization, we have developed a goal programming model to derive the priority weights from incomplete HFPRs under GDM situations. Then, on the basis of the ˇ-normalization, a multiplicative consistent hesitant fuzzy preference relation, an acceptable incomplete hesitant fuzzy preference relation, and a multiplicative consistent incomplete hesitant fuzzy preference relation have been defined. An algorithm for estimating the missing preference values in an acceptable incomplete HFPR has been proposed. Furthermore, a practical algorithm for obtaining the collective priority weight vector of the GDM problem with several complete or incomplete HFPRs has been proposed. Several numerical examples have been given to verify the proposed goal programming model and these algorithms. The main advantages ofthe proposed methods are summarized as follows:(1) The proposed methods are applicable to the GDM problem in which allthe DMs use the incomplete HFPRs to express their preferences. (2) According to the ˛-normalization, a new hesitantfuzzy goal programming model is developed to derive the priority weights from incomplete HFPRs based on multiplicative consistency. Meanwhile, by solving this model we can select the optimal preference value from all possible ones for each paired comparison of alternatives, and then obtain a corresponding FPR consisting of these optimal preferences as the reduction of the original HFPR. (3) According to the ˇ- normalization, a family of crisp FPRs with acceptable consistency can be obtained from an acceptably consistent HFPR by using a convex combination method, whose weights are found to be a form of convex combination. An interval weight generation method is also used to obtain interval weights from acceptably consistent HFPR. The obtained results show that the proposed methods are simple, effective and applicable to both consistent HFPRs and inconsistent ones generated from concrete decision-making problems, without solving any mathematical programming and complicated algebraic operation.