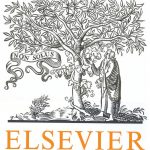
ترجمه مقاله نقش ضروری ارتباطات 6G با چشم انداز صنعت 4.0
- مبلغ: ۸۶,۰۰۰ تومان
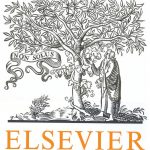
ترجمه مقاله پایداری توسعه شهری، تعدیل ساختار صنعتی و کارایی کاربری زمین
- مبلغ: ۹۱,۰۰۰ تومان
ABSTRACT
Data imputation is a common practice encountered when dealing with incomplete data. Irrespectively of the existing spectrum of techniques, the results of imputation are commonly numeric meaning that once the data have been imputed they are not distinguishable from the original data being initially available prior to imputation. In this study, the crux of the proposed approach is to develop a way of representing imputed (missing) entries as information granules and in this manner quantify the quality of the imputation process and the quality of the ensuing data. We establish a two-stage imputation mechanism in which we start with any method of numeric imputation and then form a granular representative of missing value. In this sense, the approach could be regarded as an enhancement of the existing imputation techniques. Proceeding with the detailed imputation schemes, we discuss two ways of imputation. In the first one, imputation is realized for individual variables of data sets and afterwards enhanced by the buildup of information granules. In the second approach, we are concerned with the use of fuzzy clustering, Fuzzy C-Means (FCM), which helps establish a structure in the data and then use this information in the imputation process. The design of information granules invokes the fundamentals of Granular Computing, namely a principle of justifiable granularity and an allocation of information granularity. Numeric experiments concerned with a suite of publicly available data sets offer detailed insights into the main facets of the overall design process and deliver a parametric analysis of the methods.
8. Conclusions
In this study, we discussed the problem of data imputation formulated in the new framework of Granular Computing.We showed that this two-phase approach enhances the existing techniques of imputation by making the results granular—this evidently helps tell apartthe original numeric data from those being the result ofimputation. Furthermore the approach becomes essential to quantify the quality of the imputed data by stressing their granular nature. The measure of specificity is crucial with this regard while thecoverage index characterizes the quality of the imputation process. The plots of the coverage–specificity relationships provide a general view at the nature and the quality of the process and can be sought as a certain high-level synthetic signature of the imputation process and the nature of the data. It is worth stressing that the developed concepts of granular imputation can be viewed as a follow-up process following any imputation technique, which speaks to its general nature and visible applicability of the introduced methodology. The value of the AUC measure computed on a basis of the coverage–specificity plot serves as a high-end indicator of the quality of the originally used imputation procedure.