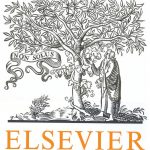
ترجمه مقاله نقش ضروری ارتباطات 6G با چشم انداز صنعت 4.0
- مبلغ: ۸۶,۰۰۰ تومان
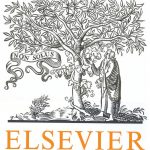
ترجمه مقاله پایداری توسعه شهری، تعدیل ساختار صنعتی و کارایی کاربری زمین
- مبلغ: ۹۱,۰۰۰ تومان
Abstract
This paper presents a novel approach for multiclass classification by fusion of KAZE and Scale Invariant Feature Transform (SIFT) features followed by Minimal Complexity Machine (MCM) as the classifier. Unlike the existing features, the paper proposes a new feature SIKA to represent characteristics of an object, as opposed to just forming a compendium of interest points in an image to represent the object characteristics. Further we append a strong and lightweight classifier, MCM to the technique. The resulting classifier easily outperforms existing techniques based on handcrafted features. Two new scores Keypoint Overlap Score (KOS) and Mean Keypoint Overlap Score (MKOS) have also been proposed as part of this work which are useful in establishing the strength of features for object representation.
8. Conclusion
This paper proposed a Fuzzy MIN-MAX combination algorithm, as a strategy to improve the writer’s soft-biometrics prediction. First, three SVM predictors associated to different data features were developed to perform writer’s gender, handedness and age range prediction. Thereafter, SVM responses are combined to improve the prediction accuracy. Comprehensive experiments using two English and Arabic handwritten text datasets, demonstrated that the proposed combination algorithm can considerably improve the prediction accuracy. Also, what we could observe is that the comparison to various combination rules as well as the state of the art, confirmed the effectiveness of this approach. Based on the results reported in this work, we believe that efficiency of the FuzzyMIN-MAXcombinationcanbedemonstratedbetter when using larger datasets. It is important to note that the combination process, handles SVM outputs provided with relevance information. This property allows the use of any kind of data features and makes it reproducible to any other pattern recognition task. To improve again our results, we intend in a future work, to investigate more robust features such as Histogram of Templates as well as new classifiers such as Artificial Immune Recognition Systems (AIRS).