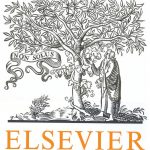
ترجمه مقاله نقش ضروری ارتباطات 6G با چشم انداز صنعت 4.0
- مبلغ: ۸۶,۰۰۰ تومان
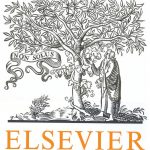
ترجمه مقاله پایداری توسعه شهری، تعدیل ساختار صنعتی و کارایی کاربری زمین
- مبلغ: ۹۱,۰۰۰ تومان
Abstract
Statistical inference, which relies on bootstrapping in partial least squares structural equation modeling (PLS-SEM), lies at the heart of developing practically relevant and academically rigorous theory. Inspection of PLS-SEM applications in European management research reveals that there is still much to be gained in terms of bootstrapping. This paper suggests several bootstrapping best practices and demonstrates how to conduct them for frequently encountered, yet often ignored, PLS-SEM situations such as the assessment of (non) direct effects, the comparison of effects, and the evaluation of the coefficient of determination.
4. Conclusion
According to Chia (2014), conducting relevant and rigorous European management research requires researchers “to imaginatively seek out ever-newer meanings to both exceptional and ordinary everyday experiences, familiar and unfamiliar happenings, and taken-for-granted conceptual formulations” (Chia, 2014, p. 686). PLS-SEM's exploratory characteristic perfectly fits this point of view and can therefore be considered a valuable data analytical approach for European management researchers. From a statistical explanatory modeling point of view, hypothesis testing is a critical element in developing relevant and rigorous theory (Shmueli & Koppius, 2011). In a PLS-SEM context, hypothesis testing relies on bootstrapping. As evidenced by the overview of PLS-SEM applications in European management research (see also Table 1), the accompanying bootstrap procedures are often suboptimal. In response to this observation, this paper provides a detailed overview of how to construct bias-correct percentile bootstrap confidence intervals and to demonstrate how these bootstrap confidence intervals can be used to test hypotheses related to frequently encountered research situations in management research. Although not directly related to a particular substantive European management research domain, this paper contributes to the European management research domain in the following ways. First, a key recommendation of our work is that in order to make better statistical inferences, which is one cornerstone of (European) management research (Cashen & Geiger, 2004; Thietart, 2001), constructing bias-corrected percentile bootstrap confidence intervals based on a large number of bootstrap samples (i.e., at least 10,000) offers a powerful approach to test a large variety of hypotheses. In terms of practical implementation, Table 3 presents a set of guidelines and minimal requirements for PLS-SEM bootstrap procedures that are relevant to researchers, editors, and reviewers alike.