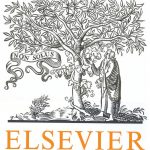
ترجمه مقاله نقش ضروری ارتباطات 6G با چشم انداز صنعت 4.0
- مبلغ: ۸۶,۰۰۰ تومان
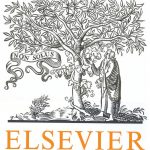
ترجمه مقاله پایداری توسعه شهری، تعدیل ساختار صنعتی و کارایی کاربری زمین
- مبلغ: ۹۱,۰۰۰ تومان
Abstract
The purpose of this study is to (1) assess the added value of information available before (i.e., leading) and after (i.e., lagging) the focal post's creation time in sentiment analysis of Facebook posts, (2) determine which predictors are most important, and (3) investigate the relationship between top predictors and sentiment. We build a sentiment prediction model, including leading information, lagging information, and traditional post variables. We benchmark Random Forest and Support Vector Machines using five times twofold cross-validation. The results indicate that both leading and lagging information increase the model's predictive performance. The most important predictors include the number of uppercase letters, the number of likes and the number of negative comments. A higher number of uppercase letters and likes increases the likelihood of a positive post, while a higher number of comments increases the likelihood of a negative post. The main contribution of this study is that it is the first to assess the added value of leading and lagging information in the context of sentiment analysis.
5. Conclusion and practical recommendations
Initially, sentiment analysis was performed mainly on review data. Recently, because of their abundance, social media data have become the main focus in the field. Despite this change in focus, our literature review shows that researchers have not yet explored the additional wealth of information that is available through social media data. Therefore, in this study we set out to (1) study the added value of leading and lagging variables for sentiment analysis, (2) determine the top predictors, (3) and explore the relationships of the top predictors with the sentiment of a post. We devised a conceptual framework to support our results. The results clearly indicate that leading and lagging variables add predictive value to established sentiment analysis models. In other words, past and future information does add value over present information. The magnitude of the differences in model performance and the consistency of these differences over all folds suggest that the results are relevant. Given that Facebook messages are informal and therefore often contain slang, irony or multi-lingual words [72], sentiment analysis is difficult based solely on text. We showed that leading and lagging variables can help to predict sentiment in this challenging environment, and our conceptual framework helped in explaining why these variables matter. The most important predictors of the most complete model were a mix of post variables (e.g., number of uppercase letters), leading variables (e.g., average number of negative comments on posts in the past) and lagging variables (e.g., number of likes) indicating that all three model components add to the predictive value of our model. We can draw several conclusions from these findings.