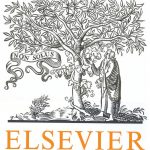
ترجمه مقاله نقش ضروری ارتباطات 6G با چشم انداز صنعت 4.0
- مبلغ: ۸۶,۰۰۰ تومان
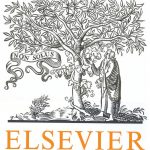
ترجمه مقاله پایداری توسعه شهری، تعدیل ساختار صنعتی و کارایی کاربری زمین
- مبلغ: ۹۱,۰۰۰ تومان
Abstract
The current trend towards integrating software agents in safety–critical systems such as drones, autonomous cars and medical devices, which must operate in uncertain environments, gives rise to the need of on-line detection of an unexpected behavior. In this work, on-line monitoring is carried out by comparing environmental state transitions with prior beliefs descriptive of optimal behavior. The agent policy is computed analytically using linearly solvable Markov decision processes. Active inference using prior beliefs allows a monitor proactively rehearsing on-line future agent actions over a rolling horizon so as to generate expectations to discover surprising behaviors. A Bayesian surprise metric is proposed based on twin Gaussian processes to measure the difference between prior and posterior beliefs about state transitions in the agent environment. Using a sliding window of sampled data, beliefs are updated a posteriori by comparing a sequence of state transitions with the ones predicted using the optimal policy. An artificial pancreas for diabetic patients is used as a representative example.
6. Final discussion
This work presents a novel probabilistic approach built upon an optimally controlled stochastic system for on-line monitoring of an agent behavior under uncertainty, which has been conceived in terms of active inference and optimal action selection. Checking if an autonomous agent behavior fulfills expectations is a key issue to guarantee safety and performance of an increasing number of autonomous agent applications such as driverless cars, drones and biomedical systems. The main problem for behavior monitoring is generating prior beliefs under the uncertainty the agent should face in its own environment. In this work, the desired behavior is modeled by a prior Gaussian distribution for state transitions, in order to verify if a given agent control policy respects its specification. The desired optimal behavior is obtained analytically using a class of Markov decision processes which are linearly solvable. Through an exponential transformation, the Bellman equation for such problems can be made linear, despite nonlinearity in the stochastic dynamical models, which facilitates applying efficient numerical methods. The availability of an optimal control policy allows simulating the desired behavior over time and comparing it with the current system performance in order to identify deviations from the desired behavior. To favor on-line monitoring, a robust metric based on surprise and twin Gaussian processes is introduced to characterize the progressive degradation in the agent behavior by quantifying the distance between the implementation and prior beliefs. A distinctive advantage of computing surprise using Gaussian processes is that the divergence from prior beliefs can be estimated not only using the expected value of state transitions but also the corresponding prediction uncertainty for optimal action selection.