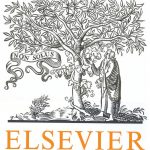
ترجمه مقاله نقش ضروری ارتباطات 6G با چشم انداز صنعت 4.0
- مبلغ: ۸۶,۰۰۰ تومان
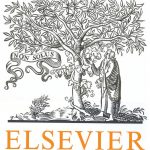
ترجمه مقاله پایداری توسعه شهری، تعدیل ساختار صنعتی و کارایی کاربری زمین
- مبلغ: ۹۱,۰۰۰ تومان
و برای خرید ترجمه کامل مقاله با فرمت ورد از دکمه های زیر استفاده نمایید.
Background A basic requirement for artificial intelligence (AI)–based image analysis systems, which are to be integrated into clinical practice, is a high robustness. Minor changes in how those images are acquired, for example, during routine skin cancer screening, should not change the diagnosis of such assistance systems.
Objective To quantify to what extent minor image perturbations affect the convolutional neural network (CNN)–mediated skin lesion classification and to evaluate three possible solutions for this problem (additional data augmentation, test-time augmentation, anti-aliasing).
Methods We trained three commonly used CNN architectures to differentiate between dermoscopic melanoma and nevus images. Subsequently, their performance and susceptibility to minor changes (‘brittleness’) was tested on two distinct test sets with multiple images per lesion. For the first set, image changes, such as rotations or zooms, were generated artificially. The second set contained natural changes that stemmed from multiple photographs taken of the same lesions.
Results All architectures exhibited brittleness on the artificial and natural test set. The three reviewed methods were able to decrease brittleness to varying degrees while still maintaining performance. The observed improvement was greater for the artificial than for the natural test set, where enhancements were minor.
Conclusions Minor image changes, relatively inconspicuous for humans, can have an effect on the robustness of CNNs differentiating skin lesions. By the methods tested here, this effect can be reduced, but not fully eliminated. Thus, further research to sustain the performance of AI classifiers is needed to facilitate the translation of such systems into the clinic.
5. Conclusions
Minor image changes, relatively inconspicuous for humans, can have an effect on the confidence and diagnosis of CNNs differentiating skin lesions. Using the methods tested here, this effect was reduced but not fully eliminated. Therefore, we would like to remind deep learning practitioners and physicians in dermatology but also in medicine in general, that brittleness needs to be explicitly targeted and overcome to facilitate translation from bench-to-bedside.
5- نتیجه گیری
تغییرات جزئی و اندک تصویر، که برای انسان ها تاحدودی نامعلوم و نامشخص هستند، می توانند روی سطح اطمینان و تشخیص شبکه های عصبی پیچشی متمایزکننده ی ضایعات پوستی تأثیر بگذارند. این تأثیر با استفاده از روش هایی که در اینجا تست و امتحان شدند کاهش یافت اما کاملا برطرف نشد. بنابراین، ما می خواهیم به متخصصان و دست اندرکاران یادگیری عمیق و پزشکان حوزه ی درماتولوژی بلکه پزشکان به طور کلی یادآوری کنیم که شکنندگی و عدم انعطاف می بایست صراحتا در رأس کار قرار گرفته و به عنوان هدف مد نظر قرار بگیرد و به منظر تسهیل انتقال از آزمایشگاه به کلینیک باید بر آن غلبه کرد.