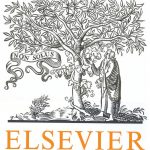
ترجمه مقاله نقش ضروری ارتباطات 6G با چشم انداز صنعت 4.0
- مبلغ: ۸۶,۰۰۰ تومان
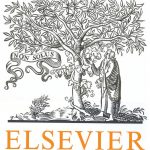
ترجمه مقاله پایداری توسعه شهری، تعدیل ساختار صنعتی و کارایی کاربری زمین
- مبلغ: ۹۱,۰۰۰ تومان
ABSTRACT
A method for the fast approximation of dexterity indices for given underwater vehicle-manipulator systems (UVMS) configurations is presented. Common underwater tasks are associated with two wellknown dexterity indices and two types of neural networks are designed and trained to approximate each one of them. The method avoids the lengthy calculation of the Jacobian, its determinant and the computationally expensive procedure of singular value decomposition required to compute the dexterity indices. It provides directly and in a considerably reduced computational time the selected dexterity index value for the given configuration of the system. The full kinematic model of the UVMS is considered and the NN training dataset is formulated by the conventional calculation of the selected dexterity indices. A comparison between the computational cost of the analytical calculation of the indices and their approximation by the two NN is presented for the validation of the proposed approach. This paper contributes mainly on broadening the applications of NN to a problem of high complexity and of high importance for UVMS high performance intervention.
5. Conclusions
In this paper common underwater tasks are associated with well-known dexterity indices and two types of neural network are designed to rapidly approximate the dexterity index given a UVMS configuration. In the design process and during the testing phase it can be observed that both FFBN and RBFN can approximate the dexterity indices within an error of no more than 2%. The dexterity is not considered as an absolute criterion on motion planning but rather as an indicator of appropriate dexterous configurations depending on the task, therefore, such a small error is acceptable for an underwater mission motion planning algorithm. The number of algebraic operations required for the analytical calculation of the selected dexterity indices was compared with number of operation required by the NN approximation. The comparison proved that the NN derived by the design process require considerably less computations than the analytical calculation. During the run-time testing procedure, the difference of the mean running time tmr , among the computational solution based on the exact form of the Jacobian and both neural network types is proven to be significant. Moreover the FFBN is proved even faster than the RBFN in every case mainly due to the less neurons utilised in the hidden layer. Even though a post-training pruning phase could benefit the RBFN by reducing the number of neurons in their hidden layer, the difference in their time performance remains quite significant. Based on the tmr performance the FFBN prove to be the best option among the networks proposed. The computationaltime reduction (10–100 times faster) yields better performance for a real-time motion planning algorithm for a UVMS that is to perform an intervention and dexterity is taken under consideration. The proposed method is applicable on any type of UVMS and could be extended in other dexterity indices too, creating a library of index approaching neural networks. The potential design of neural networks in hardware form could lead to even further computational time savings. The method could easily be extended into space robotic systems by eliminating the passivity of pitch and roll angles or even mobile systems by constraining the respective dof. In our future plans the integration of the FFBN method in an online motion planner for a UVMS is considered, in order to enhance its performance towards real-time navigation. The development of a series of NN to approach other known dexterity indices and their association with additional underwater tasks is also considered.