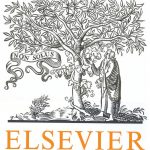
ترجمه مقاله نقش ضروری ارتباطات 6G با چشم انداز صنعت 4.0
- مبلغ: ۸۶,۰۰۰ تومان
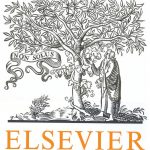
ترجمه مقاله پایداری توسعه شهری، تعدیل ساختار صنعتی و کارایی کاربری زمین
- مبلغ: ۹۱,۰۰۰ تومان
abstract
It is common practice to evaluate the strength of forecasting methods using collections of well-studied time series datasets, such as the M3 data. The question is, though, how diverse and challenging are these time series, and do they enable us to study the unique strengths and weaknesses of different forecasting methods? This paper proposes a visualisation method for collections of time series that enables a time series to be represented as a point in a two-dimensional instance space. The effectiveness of different forecasting methods across this space is easy to visualise, and the diversity of the time series in an existing collection can be assessed. Noting that the diversity of the M3 dataset has been questioned, this paper also proposes a method for generating new time series with controllable characteristics in order to fill in and spread out the instance space, making our generalisations of forecasting method performances as robust as possible. © 2016 International Institute of Forecasters. Published by Elsevier B.V. All rights reserved.
5. Conclusions
We have represented a collection of time series as points in a feature space. This has allowed us to propose several interesting analysis tools that provide insights into large collections of time series. First, we can identify unusual time series which have very different combinations of features to other time series in the collection. We can also see clustering and other structures within the feature space, showing possible subgroups of time series and regions where there are many similar time series.