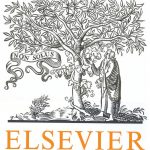
ترجمه مقاله نقش ضروری ارتباطات 6G با چشم انداز صنعت 4.0
- مبلغ: ۸۶,۰۰۰ تومان
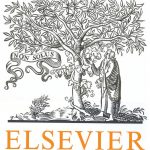
ترجمه مقاله پایداری توسعه شهری، تعدیل ساختار صنعتی و کارایی کاربری زمین
- مبلغ: ۹۱,۰۰۰ تومان
Abstract
The Internet of things (IoT) is still in its infancy and has attracted much interest in many industrial sectors including medical fields, logistics tracking, smart cities and automobiles. However as a paradigm, it is susceptible to a range of significant intrusion threats. This paper presents a threat analysis of the IoT and uses an Artificial Neural Network (ANN) to combat these threats. A multi-level perceptron, a type of supervised ANN, is trained using internet packet traces, then is assessed on its ability to thwart Distributed Denial of Service (DDoS/DoS) attacks. This paper focuses on the classification of normal and threat patterns on an IoT Network. The ANN procedure is validated against a simulated IoT network. The experimental results demonstrate 99.4% accuracy and can successfully detect various DDoS/DoS attacks.
VI. CONCLUSION AND FUTURE WORK
In this paper, we presented a neural network based approach for intrusion detection on IoT network to identify DDoS/DOS attacks. The detection was based on classifying normal and threat patterns. The ANN model was validated against a simulated IoT network demonstrating over 99% accuracy. It was able to identify successfully different types of attacks and showed good performances in terms of true and false positive rates. For future developments, more attacks shall be introduced to test the reliability of our method against attacks and improve the accuracy of the framework. Furthermore we will investigate other deeper neural networks such as the recurrent and convolutional neural network approach.