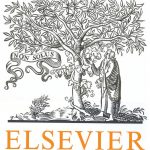
ترجمه مقاله نقش ضروری ارتباطات 6G با چشم انداز صنعت 4.0
- مبلغ: ۸۶,۰۰۰ تومان
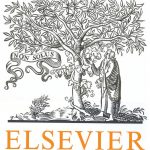
ترجمه مقاله پایداری توسعه شهری، تعدیل ساختار صنعتی و کارایی کاربری زمین
- مبلغ: ۹۱,۰۰۰ تومان
Abstract
Nonparametric mathematical models have gained a very massive attention in the last two decades in solving regression problem. The application of soft computing methodologies produced a very remarkable assistance to human abilities especially in solving nonlinear and non-stationary engineering problems. The current article investigates the utility of k-nearest neighbor (k-nn) approach in predicting ultimate bearing capacity of shallow foundation. The inspected application involves an experimental data set of foundation dimension and soil properties that suggested and calculated via manual computational methods. The predictive model is established using dimensional shallow foundation, and soil properties are an inputs variable, whereas the bearing capacity is the output variable. For the purpose of comparison and evaluating the modeling accuracy, multiple linear regression (MLR) model is chosen to diagnose the result accuracies. Couple of statistical indicators are utilized to exhibit the performance criteria of the predictive model including coefficient of determination (r 2 ), degree of agreement (d), root-mean-square error (RMSE) and mean absolute percentage error (MAPE). The results exhibited a very representable and high accuracies of the investigated k-nn model vis-a`-vis MLR. For instance, the RMSE and MAPE were enhanced by 24 and 17%, respectively. In addition, the findings indicated that k-nn provides an accurate and reliable alternative predictive model to the manual computational methods.
5 Conclusions
In this research, the potential capability of nonparametric mathematical model called k-nearest neighbor was inspected to predict the ultimate bearing capacity (qu) of shallow foundation. The modeling was developed based on geometry parameters (i.e., foundation dimensions) and soil properties; on the other hand, the target parameter was qu. The motivation of this study is to explore an alternative intelligent model to predict qu with less complication, save execution time and produce high level of accuracy. k-nn was verified against common regression model that has been applied for the application by numerous scholars which is MLR. In general, the results of this study indicated that k-nn model provided reliable predictive model for the geotechnical engineering, particularly to predict ultimate bearing capacity of shallow foundation. In more numerical details, the augmentation of the absolute error measurements between k-nn and MLR models was 24 and 17% for RMSE and MAPE, respectively.