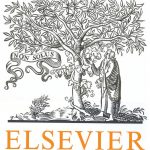
ترجمه مقاله نقش ضروری ارتباطات 6G با چشم انداز صنعت 4.0
- مبلغ: ۸۶,۰۰۰ تومان
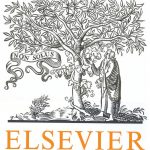
ترجمه مقاله پایداری توسعه شهری، تعدیل ساختار صنعتی و کارایی کاربری زمین
- مبلغ: ۹۱,۰۰۰ تومان
TIHM INFRASTRUCTURE
This section describes the design and implementation of our IoT architecture, which is capable of combining different, yet complementary, technologies to develop novel functionalities. Our system collects real-time data from multiple publishers and delivers the data to the TIHM back-end system. At this stage, an advanced analytics tool is used to process the integrated data and generate insights and notifications based on the status of patients. Note that clinical knowledge and experience has been used to set the parameters for alerting and notifications. The high-level overview of the interactions in TIHM architecture is presented in Figure 1. As shown, it is composed of four main parts: • sensors installed at homes; • SMEs’ back-end servers; • the TIHM back-end system, including the storage and analysis servers; and • the user interface for data visualization and management.
CONCLUSION
It is estimated that there will be one million people with dementia in the UK by 2025.1 Beyond those directly affected, dementia has a much wider impact on carers, families, and healthcare services, with social isolation being a key confounding factor. TIHM aims to develop innovative living environments that empower people with dementia and their carers to enjoy better health and quality of life, with reduced dependence on institutional care. One of the key aspects of this project is that user-led design principles have been at the heart of developing the system, allowing us to draw expertise and experience from people with dementia, carers, clinicians, academics, and industry partners. Furthermore, we focus on integrating various data from different providers. In this regard, we have developed a flexible system to integrate the existing heterogeneous data and facilitate the easy integration of other publishers for further expansion because of our semantic interoperability. In addition, we have applied advanced algorithms to analyze the acquired multimodal data, extract meaningful information, and generate online notifications for caregivers. One of the key features of the machine-learning and data analytics algorithms in TIHM is that they combine environmental and physiological data to learn and discover changes in patients’ health and well-being. At this point, a number of algorithms have been developed to • learn the patients’ daily patterns and find possible pattern deviations, • detect if patients are agitated or irritated, and • detect the possibility of UTIs.