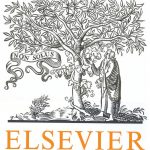
ترجمه مقاله نقش ضروری ارتباطات 6G با چشم انداز صنعت 4.0
- مبلغ: ۸۶,۰۰۰ تومان
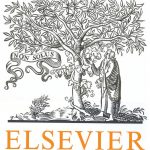
ترجمه مقاله پایداری توسعه شهری، تعدیل ساختار صنعتی و کارایی کاربری زمین
- مبلغ: ۹۱,۰۰۰ تومان
Abstract
In recent years graph pattern mining took a prominent role in knowledge discovery in many scientific fields. From Web advertising to biology and finance, graph data is ubiquitous making pattern-based graph tools increasingly important. When it comes to financial settings, data is very complex and although many successfully approaches have been proposed often they neglect the intertwined economic risk factors, which seriously affects the goodness of predictions. In this paper, we posit that financial risk analysis can be leveraged if structure can be taken into account by discovering financial motifs. We look at this problem from a graph-based perspective in two ways, by considering the structure in the inputs, the graphs themselves, and by taking into account the graph embedded structure of the data. In the first, we use gBoost combined with a substructure mining algorithm. In the second, we take a subspace learning graph embedded approach. In our experiments two datasets are used: a qualitative bankruptcy data benchmark and a real-world French database of corporate companies. Furthermore, we propose a graph construction algorithm to extract graph structure from feature vector data. Finally, we empirically show that in both graph-based approaches the financial motifs are crucial for the classification, thereby enhancing the prediction results.
Conclusion and Future Work
The combination of the formalism of graphs with a powerful frequent pattern mining algorithm such as gBoost evidenced that the structure is able to effectively capture knowledge essential to attain good predictions in financial settings. In this work we developed an algorithm for graph construction on the grounds of the binary relationships found on qualitative data from the financial credit risk problem. We used gBoost classifier to mine specific sampled graphs that are able to predict the samples category in either bankrupt or non-bankrupt. Furthermore, when large-scale historical data is available the data can be cast into an embedded graph. Once we obtain compact representations of the firms behavior, the subspace learning procedure can effectively be performed in the lower dimensional subspace with an SVM. Both methodologies can find the graph motifs in data which are able to foster better predictions using as the experimental datasets respectively a qualitative data benchmark from UCI Machine Learning Repository and a real-world French database of corporate companies. The experimental results empirically demonstrated by using structural approaches the performance results can be enhanced in terms of prediction accuracy in particular if graphs to cast data are carefully built. While most of the approaches consider supplying known values of required input variables, in the pattern mining approach the structural component is also taken into account making the model effective and robust. It can partly be used herein to shed light on how both approaches incorporate more knowledge through the graph component for better exposure of credit risk financial problems. Another reason for using graph pattern mining is the easiness of visualizing financial data in the big data era. In summary, graph mining has promising advantages on distributed graph algorithms, graph data visualization and easiness to deal with big data.