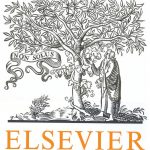
ترجمه مقاله نقش ضروری ارتباطات 6G با چشم انداز صنعت 4.0
- مبلغ: ۸۶,۰۰۰ تومان
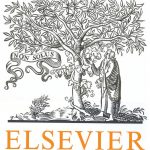
ترجمه مقاله پایداری توسعه شهری، تعدیل ساختار صنعتی و کارایی کاربری زمین
- مبلغ: ۹۱,۰۰۰ تومان
Abstract
Ensemble is a widely used technique to improve the predictive performance of a learning method by using several competing expert systems. In this study, we propose a new ensemble combination scheme using a latent consensus function that relates each predictor to the other. The proposed method is designed to adapt and self-correct weights even when a number of expert systems malfunction and become corrupted. To compare the performance of the proposed method with existing methods, experiments are performed on simulated data with corrupted outputs as well as on real-world data sets. Results show that the proposed method is effective and it improves the predictive performance even when a number of individual classifiers are malfunctioning.
6. Conclusion
In this study, we have proposed a novel approach for ensemble combination scheme using a latent consensus function that relates individual predictors. Our basic idea is that the predicted value of individual predictors is composed of the reflection of the real function value and a specific error term. According to this assumption, we determine weights for the ensemble combination using a separate training algorithm. We have presented a comprehensive evaluation ofthe proposed method on simulated data set as well as real world data sets using neural networks and decision trees as base models. The experimental results show that the proposed method further improves its prediction performance by using self-correction for the malfunctioning base learners. By analyzing the results of corrupted toy data sets, we have shown that an ensemble can adjust weights by detecting the corrupt predictors in the learning process. Therefore, the proposed method can improve its performance even when a number of individual predictor outputs are corrupted. Future research will investigate two aspects. First, the predictor selection ability of the proposed method can be enhanced. Second, the effectiveness of the proposed method can be enhanced when different base learners like a learning model that uses the concepts of unsupervised models for supervised tasks [13,16,15,22,21,23], data sizes, and distributions are used.