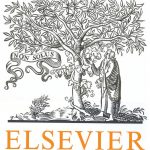
ترجمه مقاله نقش ضروری ارتباطات 6G با چشم انداز صنعت 4.0
- مبلغ: ۸۶,۰۰۰ تومان
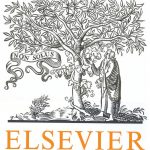
ترجمه مقاله پایداری توسعه شهری، تعدیل ساختار صنعتی و کارایی کاربری زمین
- مبلغ: ۹۱,۰۰۰ تومان
ABSTRACT
Human activity recognition is an active area of research in Computer Vision. One of the challenges of activity recognition system is the presence of noise between related activity classes along with high training and testing time complexity of the system. In this paper, we address these problems by introducing a Robust Least Squares Twin Support Vector Machine (RLS-TWSVM) algorithm. RLS-TWSVM handles the heteroscedastic noise and outliers present in activity recognition framework. Incremental RLS-TWSVM is proposed to speed up the training phase. Further, we introduce the hierarchical approach with RLSTWSVM to deal with multi-category activity recognition problem. Computational comparisons of our proposed approach on four well-known activity recognition datasets along with real world machine learning benchmark datasets have been carried out. Experimental results show that our method is not only fast but, yields significantly better generalization performance and is robust in order to handle heteroscedastic noise and outliers.
5. Conclusions
In this paper, we have proposed an improved and robust version of LS-TWSVM called RLS-TWSVM to incorporate the effect of outliers and heteroscedastic noise involved due to closely related activity classes in human activity recognition framework. We introduced the Incremental RLS-TWSVM to deal with large datasets in activity recognition problem. We introduce the idea of activity class hierarchy to use the idea of relatedness among activities. The proposed framework addresses the problem of heteroscedastic noise, high training time and unbalanced dataset. In our experiments, we have used feature descriptor based on silhouette and optic flow. To investigate the validity and the effectiveness of RLS-TSVM, experiments were conducted on the well-known Weizmann, UIUC1,IXMAS and UMD humanactiondatasets. Experiments have also been carried out on several UCI datasets. The results showed the out-performance of proposed RLS-TWSVM over other state-of-art methods. In this paper, we have considered datasets where only one actor is performing a single action. However, it would be interesting to explore the application of the proposed approach to such scenarios where actor-actor and object-actor interaction is involved. This would further open the window to explore other trajectory and depth based features.