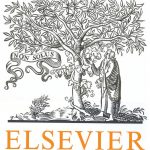
ترجمه مقاله نقش ضروری ارتباطات 6G با چشم انداز صنعت 4.0
- مبلغ: ۸۶,۰۰۰ تومان
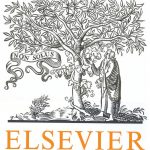
ترجمه مقاله پایداری توسعه شهری، تعدیل ساختار صنعتی و کارایی کاربری زمین
- مبلغ: ۹۱,۰۰۰ تومان
abstract
Of late, neural networks and Multiple Instance Learning (MIL) are both attractive topics in the research areas related to Artificial Intelligence. Deep neural networks have achieved great successes in supervised learning problems, and MIL as a typical weakly-supervised learning method is effective for many applications in computer vision, biometrics, natural language processing, and so on. In this article, we revisit Multiple Instance Neural Networks (MINNs) that the neural networks aim at solving the MIL problems. The MINNs perform MIL in an end-to-end manner, which take bags with a various number of instances as input and directly output the labels of bags. All of the parameters in a MINN can be optimized via back-propagation. Besides revisiting the old MINNs, we propose a new type of MINN to learn bag representations, which is different from the existing MINNs that focus on estimating instance label. In addition, recent tricks developed in deep learning have been studied in MINNs; we find deep supervision is effective for learning better bag representations. In the experiments, the proposed MINNs achieve state-of-the-art or competitive performance on several MIL benchmarks. Moreover, it is extremely fast for both testing and training, for example, it takes only 0.0003 s to predict a bag and a few seconds to train on MIL datasets on a moderate CPU.
6. Conclusion
In this study, we revisit the problem of end-to-end learning of MINNs and propose a series of novel MINNs with the state-of-theart performance. Different from the existing MINNs, our method focuses on bag-level representation learning instead of instancelevel label estimating. Experiments show that our bag-level networks show superior results on several MIL benchmarks compared with the instance-level networks. Moreover, we integrate the most popular deep learning tricks (deep supervision and residual connections) into our networks, which can boost the performance further. Moreover, our method only takes about 0.0003 s for testing (forward) and 0.0008 s for training (backward) per bag, which is very efficient.