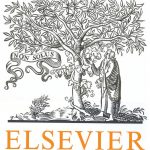
ترجمه مقاله نقش ضروری ارتباطات 6G با چشم انداز صنعت 4.0
- مبلغ: ۸۶,۰۰۰ تومان
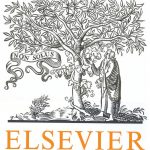
ترجمه مقاله پایداری توسعه شهری، تعدیل ساختار صنعتی و کارایی کاربری زمین
- مبلغ: ۹۱,۰۰۰ تومان
ABSTRACT
Energy system optimization models (ESOMs) have been used extensively in providing insights to decision makers on issues related to climate and energy policy. However, there is a concern that the uncertainties inherent in the model structures and input parameters are at best underplayed and at worst ignored. Compared to other types of energy models, ESOMs tend to use scenarios to handle uncertainties or treat them as a marginal issue. Without adequately addressing uncertainties, the model insights may be limited, lack robustness, and may mislead decision makers. This paper provides an in-depth review of systematic techniques that address uncertainties for ESOMs. We have identified four prevailing uncertainty approaches that have been applied to ESOM type models: Monte Carlo analysis, stochastic programming, robust optimization, and modelling to generate alternatives. For each method, we review the principles, techniques, and how they are utilized to improve the robustness of the model results to provide extra policy insights. In the end, we provide a critical appraisal on the use of these methods.
Discussion and conclusion
The value of energy system modelling is on highlighting policy implications rather than providing absolute numbers - providing insights rather than answers. Compared to conventional scenario analysis, assessing uncertainties in a systematic manner helps improve the robustness of results and provide additional insights associated with multiple outcomes. In this paper, we carried out a comprehensive review of uncertainty techniques that have been applied to ESOM models: Monte Carlo analysis, stochastic programming, robust optimization, and modelling to generate alternatives. A key finding arising from this review is that each of the four uncertainty analysis techniques has its own focus, advantages and limitations, and informs different aspects of decision-making. Choosing a specific uncertainty technique should involve consideration of issues such as data availability, the uncertainty space to be covered, and the type of policy questions to be answered. Fig. 4 provides guidance and recommendations for modellers in the form of a flow chart that summarizes the key policy insights for each technique and a basis for selecting which uncertainty technique to use. It is also worth noting that uncertainty analysis approaches are not mutually exclusive and should be used in a complementary manner to provide well-rounded analysis. MCA can be applied when information on probability distributions could be obtained through existing studies or expert elicitation. In addition to quantifying the feasibility in reaching policy targets and identifying robust technologies, MCA can also be run in tandem with GSA to map the relationships between inputs and outputs, which improves model transparency and unpacks model structure. As the only approach for sequential decision-making, stochastic programming is best used when the number of uncertain sources under concern is small. It can be used to provide a single optimal hedging strategy that can help guide near-term action. Such an approach avoids the issue with multiscenario approaches, where the scenario ensemble may leave the decision makers in a quandary. Robust optimization is a computationally efficient approach for handling uncertainties associated with a large set of parameters while requiring minimal information on the distribution of uncertain parameters.