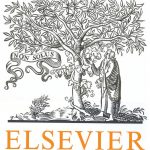
ترجمه مقاله نقش ضروری ارتباطات 6G با چشم انداز صنعت 4.0
- مبلغ: ۸۶,۰۰۰ تومان
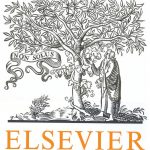
ترجمه مقاله پایداری توسعه شهری، تعدیل ساختار صنعتی و کارایی کاربری زمین
- مبلغ: ۹۱,۰۰۰ تومان
Abstract
A new paradigm for emergency networking is envisioned to enable reliable and high data-rate wireless multimedia communication among public safety agencies in licensed spectrum while causing only acceptable levels of disruption to incumbent network communication. The novel concept of mission policies, which specify the Quality of Service (QoS) requirements of the incumbent networks as well as of the emergency networks involved in rescue and recovery missions, is introduced. The use of mission policies, which vary over time and space, enables graceful degradation in the QoS of the incumbent networks (only when necessary) based on mission policy specifications. A Multi-Agent Reinforcement Learning (MARL)-based cross-layer communication framework, “RescueNet,” is proposed for self-adaptation of nodes in emergency networks based on this new paradigm. In addition to addressing the research challenges posed by the non-stationarity of the problem, the novel idea of knowledge sharing among the agents of different ages (either bootstrapping or selective exploration strategies or both) is introduced to improve significantly the performance of the proposed solution in terms of convergence time and conformance to the mission policies.
5. Conclusion and future work
We introduced a policy- and learning-based paradigm for emergency networking in conditionally auctioned licensed spectrum. The concept of mission policies, which specify the Quality of Service (QoS) for emergency as well as for incumbent network traffic, is envisioned. Our paradigm for emergency networking represents a shift from the established primary–secondary model (which uses fixed priorities) and enables graceful degradation in the QoS of incumbent networks based on mission-policy specifications. We developed a Multi-Agent Reinforcement Learning (MARL)-based communication framework, RescueNet, for realizing this new paradigm. The proposed solution can go beyond the emergency scenario and has the potential to enable cognitive ad hoc network operation in any frequency band, licensed or unlicensed. The performance of RescueNet in terms of convergence and policy conformance is verified using simulations on ns-3. Our future work includes Inverse Reinforcement Learning (IRL) for the reward function. As the scalar reward function does not provide optimal performance in dynamically changing environment, we will study the IRL problem to optimize the reward function when a priori knowledge is available on the fly. The IRL problem consists in finding a reward function that can explain observed behavior. We will focus initially on the setting in which the complete prior knowledge and mission policy are given; then, we will find new methods to choose among optimal reward functions as multiple possible reward functions may exist.