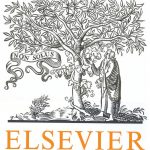
ترجمه مقاله نقش ضروری ارتباطات 6G با چشم انداز صنعت 4.0
- مبلغ: ۸۶,۰۰۰ تومان
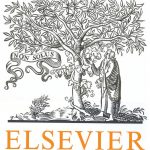
ترجمه مقاله پایداری توسعه شهری، تعدیل ساختار صنعتی و کارایی کاربری زمین
- مبلغ: ۹۱,۰۰۰ تومان
ABSTRACT
In this paper, a novel CMOQPSO algorithm is proposed, in which cultural evolution mechanism is introduced into quantum-behaved particle swarm optimization (QPSO) to solve multiobjective environmental/economic dispatch (EED) problems. There are growing concerns about the ability of QPSO to handle multiobjective optimization problems. Two important issues in extending QPSO to multiobjective context are the construction of exemplar positions for each particle and the maintenance of population diversity. In the proposed CMOQPSO, one particle is measured for multiple times at each iteration in order to enhance its global searching ability. Belief space, which is based on cultural evolution mechanism and contains different types of knowledge extracted from the particle swarm, is adopted to generate global best positions for the multiple measurements of each particle. Moreover, to maintain population diversity and avoid premature, a novel local search operator, which is based on the knowledge in belief space, is proposed in this paper. CMOQPSO is compared with several state-of-art algorithms and tested on EED systems with 6 and 40 generators respectively. The comparative results demonstrate the effectiveness of the proposed algorithm.
5. Concluding remarks
In this paper, a multiobjective QPSO algorithm, called CMOQPSO, was adopted to solve EED problems. CMOQPSO has introduced cultural evolution mechanism into traditional QPSO to solve multiobjective optimization problems. In CMOQPSO,the evolution of the particles is guided by belief space which contains different types of knowledge extracted from the particle swarm. Another difference between CMOQPSO and the traditional QPSO is that each particle in CMOQPSO is measured for multiple times. For each particle, the global best positions of the multiple measurements are obtained according to the knowledge in belief space. Moreover, a local search operator is proposed to enhance the particles’ ability of jumping out of local optima. In order to show the effectiveness of the proposed algorithm, CMOQPSO is tested on EED systems with 6 and 40 generators respectively. The performance of CMOQPSO is compared with the other algorithms from different aspects. The comparative results have shown the effectiveness and superiority of CMOQPSO. However, in CMOQPSO each particle was measured for multiple times, this may lead to a relatively slower convergence speed in comparison with some other algorithms. This problem will be our future work of this paper.