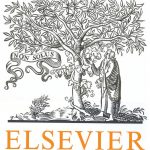
ترجمه مقاله نقش ضروری ارتباطات 6G با چشم انداز صنعت 4.0
- مبلغ: ۸۶,۰۰۰ تومان
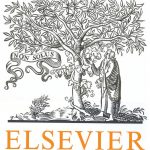
ترجمه مقاله پایداری توسعه شهری، تعدیل ساختار صنعتی و کارایی کاربری زمین
- مبلغ: ۹۱,۰۰۰ تومان
Abstract
The presence of a rich set of embedded sensors on mobile devices has been propelling various sensing applications regarding individual activities and their surrounding environment, and these persuasive sensing-capable mobile devices are pushing the new paradigm of Mobile Crowd Sensing (MCS) from sketch to reality. MCS aims to outsource sensing data collection to Mobile Device Owner (MDO) and it could revolutionize the conventional ways of sensing data collection and processing. Nonetheless, the widespread deployment of MCS gives rise to the privacy concerns from both the MDOs and the Sensing Service Consumers (SSC), especially in the case where MCS relies on untrustworthy third-party infrastructures. This paper proposes three protocols to address the privacy issues of MCS in ad hoc network without depending on any third-parties. It first presents Privacy-Preserving Summation (PPS) protocol to protect the privacy of the SSCs. Next, it puts forward Privacy-Preserving Difference Rank Computation (PPDRC) protocol to ensure the privacy of the MDOs. Finally, it proposes Approximate K-Nearest Neighbor with Privacy Preservation(AKN2P2) to approximately identify the k-nearest neighbors without privacy leaks of both the MDOs and the SSCs. The performance evaluations demonstrate the computation overhead in different settings.
8. Conclusion
In this paper, we studied various ways to protect the privacy of the SSC and the MDOs in different MCS scenarios. We first proposed PPSQ to secure the privacy of the summation query of the SSC regarding the real target MDOs. Next, we put forward PPDRC to protect the data privacy of the MDOs to retrieve the difference rank in the multi-party computation process. As the signs of polynomials can be derived without disclosing the numeric values of the data records of the involved MDOs, given a baseline value d, the difference between the sensing reading of any MDO and d can be compared against a designated proportion of the summation of sensing reading differences of a group of MDOs without revealing the values of d and any sensing readings. Subsequently, we elaborated how to identify K-nearest neighbors around the POO issued by the SSC with minimum error, while keeping the POO and the locations of all involved MDOs secrete. In this solution, all the possible distance values between the PPO and each MDO location are included in a distance range, and all the MDOs agree on the privacy window of the smallest size such that the privacy level regarding the distances of some MDOs is still acceptable even in the worst case. Security analysis are given and the performance evaluations are done at the end.