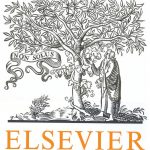
ترجمه مقاله نقش ضروری ارتباطات 6G با چشم انداز صنعت 4.0
- مبلغ: ۸۶,۰۰۰ تومان
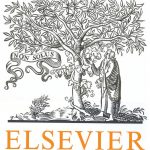
ترجمه مقاله پایداری توسعه شهری، تعدیل ساختار صنعتی و کارایی کاربری زمین
- مبلغ: ۹۱,۰۰۰ تومان
Abstract
Can the sentiment contained in tweets serve as a meaningful proxy to predict match outcomes and if so, can the magnitude of outcomes be predicted based on a degree of sentiment? To answer these questions we constructed the CentralSport system to gather tweets related to the twenty clubs of the English Premier League and analyze their sentiment content, not only to predict match outcomes, but also to use as a wagering decision system. From our analysis, tweet sentiment outperformed wagering on odds-favorites, with higher payout returns (best $2704.63 versus odds-only $1887.88) but lower accuracy, a trade-off from non-favorite wagering. This result may suggest a performance degradation that arises from conservatism in the odds-setting process, especially when three match results are possible outcomes. We found that leveraging a positive tweet sentiment surge over club average could net a payout of $3011.20. Lastly, we found that as the magnitude of positive sentiment between two clubs increased, so too did the point spread; 0.42 goal difference for clubs with a slight positive edge versus 0.90 goal difference for an overwhelming difference in positive sentiment. In both these cases, the cultural expectancy of positive tweet dominance within the twitter-base may be realistic. These outcomes may suggest that professional odds-making excessively predicts non-positive match outcomes and tighter goal spreads. These results demonstrate the power of hidden information contained within tweet sentiment and has predictive implications on the design of automated wagering systems.
7. Conclusions and future directions
From our study we found that crowdsourced sentiment can be a better predictor of match outcomes than crowdsourced odds. In looking at accuracy and payout, the crowdsourced odds-only (Baseline) approach had the highest accuracy versus the eight sentiment models tested. However, in terms of payout, five of the eight sentiment models had higher returns (Subjective Negative $46.82, Objective Negative $58.65, Objective Positive $382.67, All Objective $816.75 and All Negative $726.65). The three models with returns less than Baseline were all clustered around Subjective Positive (Subjective Positive − $1064.50, All Subjective −$2083.42 and All Positive −$179.36). Of the three, only All Subjective lost money, −$195.54. We believe that crowdsourced sentiment was better at identifying longshot wagers as evidenced by five of the sentiment models. For the other three we found the subjective positiveness harmed the results and believe this to be a reaction to events and overconfidence in club performance, rather than rational prescriptive observation transcribed to tweet sentiment. In looking specifically at the models of All Positive and All Negative sentiments and evaluating the surge/drop of match sentiment versus their club average, we found that this technique led to higher accuracy and payouts for the All Positive model (53.28% accuracy and $3011.20 payout). Conversely, All Negative showed a marked decline in performance (27.87% accuracy and $315.17 payout). This result indicates that positive surges (above average club levels) in tweet sentiment generally lead to better match predictability. Tweet authors recognize some factor in their clubs' performance and express it through tweet sentiment. While we expected a similar result with negative sentiment (e.g., recognize something wrong with the club and expect a loss), this was not the case. However, upon a deeper analysis it appears that tweeters from weaker clubs were purposefully injecting negative sentiment into the feeds of their stronger opponents.