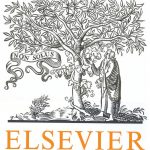
ترجمه مقاله نقش ضروری ارتباطات 6G با چشم انداز صنعت 4.0
- مبلغ: ۸۶,۰۰۰ تومان
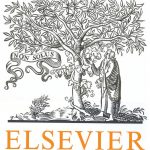
ترجمه مقاله پایداری توسعه شهری، تعدیل ساختار صنعتی و کارایی کاربری زمین
- مبلغ: ۹۱,۰۰۰ تومان
Abstract
In computer vision, significant advances have been made in recent years on object recognition and detection with the rapid development of deep learning, especially deep convolutional neural networks (CNN). The majority of deep learning methods for object detection have been developed for large objects and their performances on small-object detection are not very good. This paper contributes to research in low-resolution small-object detection by evaluating the performances of leading deep learning methods for object detection using a common dataset, which is a new dataset for bird detection, called Little Birds in Aerial Imagery (LBAI), created from real-life aerial imagery data. LBAI contains birds with sizes ranging from 10px to 40px. In our experiments, some of the best deep learning architectures were implemented and applied to LBAI, which include object detection techniques such as YOLOv2, SSH, and Tiny Face, in addition to small instance segmentation techniques including U-Net and Mask R-CNN. Among the object detection methods, experimental results demonstrated that SSH performed the best for easy cases, whereas Tiny Face performed the best for hard cases, i.e. where a cluttered background makes detecting birds difficult. Among small instance segmentation methods, experimental results revealed U-Net achieved slightly better performance than Mask R-CNN.
VI. SUMMARY
In this paper, we have presented a new aerial imagery dataset based on real-life images including waterfowl and other water birds in wetlands around the Midwest. Different from most of the existing datasets, the new LBAI dataset contains small birds of sizes ranging from 10px to 40px. Several state-of-the-art deep learning object detection and instance segmentation techniques have been applied to the LBAI database and obtained a range of performance results. Among object detection methods, SSH performed the best on the easy cases, while Tiny Face achieved the best accuracy on the hard cases. Between instance segmentation methods, U-Net achieved better performance than Mask R-CNN. These results are useful for identifying the strengths and weaknesses of existing methods and the development of future methods with improved performance.