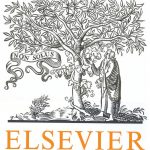
ترجمه مقاله نقش ضروری ارتباطات 6G با چشم انداز صنعت 4.0
- مبلغ: ۸۶,۰۰۰ تومان
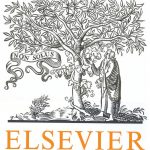
ترجمه مقاله پایداری توسعه شهری، تعدیل ساختار صنعتی و کارایی کاربری زمین
- مبلغ: ۹۱,۰۰۰ تومان
Abstract
One of the most complex physiological systems whose modeling is still an open study is the respiratory control system where different models have been proposed based on the criterion of minimizing the work of breathing (WOB). The aim of this study is twofold: to compare two known models of the respiratory control system which set the breathing pattern based on quantifying the respiratory work; and to assess the influence of using direct-search or evolutionary optimization algorithms on adjustment of model parameters. This study was carried out using experimental data from a group of healthy volunteers under CO2 incremental inhalation, which were used to adjust the model parameters and to evaluate how much the equations of WOB follow a real breathing pattern. This breathing pattern was characterized by the following variables: tidal volume, inspiratory and expiratory time duration and total minute ventilation. Different optimization algorithms were considered to determine the most appropriate model from physiological viewpoint. Algorithms were used for a double optimization: firstly, to minimize the WOB and secondly to adjust model parameters. The performance of optimization algorithms was also evaluated in terms of convergence rate, solution accuracy and precision. Results showed strong differences in the performance of optimization algorithms according to constraints and topological features of the function to be optimized. In breathing pattern optimization, the sequential quadratic programming technique (SQP) showed the best performance and convergence speed when respiratory work was low. In addition, SQP allowed to implement multiple non-linear constraints through mathematical expressions in the easiest way. Regarding parameter adjustment of the model to experimental data, the evolutionary strategy with covariance matrix and adaptation (CMA-ES) provided the best quality solutions with fast convergence and the best accuracy and precision in both models. CMAES reached the best adjustment because of its good performance on noise and multi-peaked fitness functions. Although one of the studied models has been much more commonly used to simulate respiratory response to CO2 inhalation, results showed that an alternative model has a more appropriate cost function to minimize WOB from a physiological viewpoint according to experimental data.
7. Discussion and conclusion
7.1. Optimization problem Two approaches for the respiratory control system modeling based on simultaneous optimization of ventilation and breathing pattern, which were called in this study RSM1 and RSM2, have been analyzed [9]. Such optimization was performed by minimizing a respiratory cost function (J) that reflects the balance of chemical (Jc) and mechanical (Jm) costs of breathing. Differences between both approaches were determined by the equations proposed to quantify Jm during inspiratory and expiratoryphases (Eqs.(4a),(5a), (4b) and (5b) respectively), which in turn represent estimates ofthe mechanical work of breathing (WOB). Although in this model, two equation sets were proposed to quantify the mechanical cost, RSM2 was discarded in [9] because it always led to an impulsive inspiratory pressure profile with an extremely small inspiratory duty cycle; this behavior had been also noted by other researchers [83,75]. However, in this study with the used optimization techniques was possible to adjust the model parameters in order to obtain a response with a realistic behavior (see Figs. 3 and 10). The fitting of model parameters involved two nested optimizations: the optimization of breathing pattern by fitting x = [t1, t2, a1, a2, ] and the adjustment of model responses to experimental data by fitting = [1, 2, n]. The former was performed breath to breath by minimizing J, whereas the latter was performed by minimizing PE in all populations.