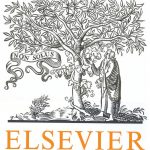
ترجمه مقاله نقش ضروری ارتباطات 6G با چشم انداز صنعت 4.0
- مبلغ: ۸۶,۰۰۰ تومان
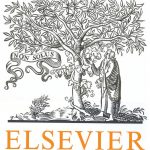
ترجمه مقاله پایداری توسعه شهری، تعدیل ساختار صنعتی و کارایی کاربری زمین
- مبلغ: ۹۱,۰۰۰ تومان
abstract
The formation of crystals from solutions plays a key role in various industrial applications. In this study, a new approach is presented into the optimal control of batch cooling crystallizers through a genetic algorithm. The Population balance is formularized for a typical batch crystallizer. The objective functions considered here are related to quality of products at the end of the batch. These functions are objective function of maximum mean weight size, closeness to the specified value and minimum coefficient of variation. By using an optimization algorithm (genetic algorithm), the minimum and maximum values of the objective function the input temperature parameter are obtained. The obtained results show that various trajectories can be used for cooling batch crystallizer based on objective functions. This method is applied for the potassium-nitrate system.
7. Conclusion
In this work, the batch cooling crystallization procedure is investigated to develop an appropriate optimization strategy. The influence of the solubility is studied to characterize the temperature of batch cooling crystallization. Temperature control policy is necessary in the batch cooling crystallization to provide a suitable crystal size distribution for the product through the optimization. Hence, batch cooling crystallization is initially modeled. Then, objective functions are used to optimize the temperature of crystallization through a genetic algorithm. Maximum mean size, closeness to the desired value (desired mean weight size) and the minimum coefficient of variation are applied to optimize the temperature profile by the genetic algorithm. Results show that the desired value objective function that is presented in the first time is the best objective function. The mean relative error of this objective function is lower than other functions that presented in this study.