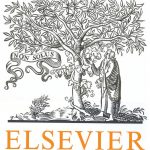
ترجمه مقاله نقش ضروری ارتباطات 6G با چشم انداز صنعت 4.0
- مبلغ: ۸۶,۰۰۰ تومان
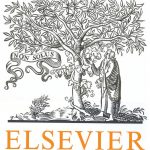
ترجمه مقاله پایداری توسعه شهری، تعدیل ساختار صنعتی و کارایی کاربری زمین
- مبلغ: ۹۱,۰۰۰ تومان
Abstract
Nonlinear groundwater flow models have the propensity to be overly complex leading to burdensome computational demands. Reduced modeling techniques are used to develop an approximation of the original model that has smaller dimensionality and faster run times. The reduced model proposed is a combination of proper orthogonal decomposition (POD) and the discrete empirical interpolation method (DEIM). Solutions of the full model (snapshots) are collected to represent the physical dynamics of the system and Galerkin projection allows the formulation of a reduced model that lies in a subspace of the full model. Interpolation points are added through DEIM to eliminate the reduced model's dependence on the dimension of the full model. POD is shown to effectively reduce the dimension of the full model and DEIM is shown to speed up the solution by further reducing the dimension of the nonlinear calculations. To show the concept can work for unconfined groundwater flow model, with added nonlinear forcings, one-dimensional and two-dimensional test cases are constructed in MODFLOW-OWHM. POD and DEIM are added to MODFLOW as a modular package. Comparing the POD and the POD-DEIM reduced models, the experimental results indicate similar reduction in dimension size with additional computation speed up for the added interpolation. The hyper-reduction method presented is effective for models that have fine discretization in space and/or time as well as nonlinearities with respect to the state variable. The dual reduction approach ensures that, once constructed, the reduced model can be solved in an equation system that depends only on reduced dimensions.
5. Conclusion
A traditional model reduction technique for groundwater flow has been combined with an interpolation scheme to further reduce nonlinear components. The result is a reduced model of an uncon- fined flow equation that can be solved entirely in the reduced dimension with no dependence on the original, full model complexity. This additional approximation allows for faster calculations of nonlinear operations at each time step while sacrificing a tractably small amount of accuracy. As simulation models get more complex, with finer discretization, larger domains, and more nonlinear processes, faster calculations become more important. The combined model reduction approach with POD and DEIM greatly improves a modeler’s ability to obtain solutions quickly. The results from the two test problems show a two to three orders of dimension reduction. A key advantage of the POD-DEIM model is that nonlinear operations are carried in the reduced space. The faster overall simulation times are critical when embedding within or linking the model to any form of optimization (e.g., parameter estimation, experimental design, resource allocation) or extensive uncertainty analysis (e.g., Monte Carlo). While more and more optimization algorithms are taking advantage of parallel computing power, long simulation runtimes still inhibit the attainment of optimal solutions in reasonable amounts of time. Therefore, reduced models such as those developed with POD-DEIM can be used within parallel architectures to facilitate searching very large feasible regions— regions with dimensions so large that they would otherwise be impossible to explore.