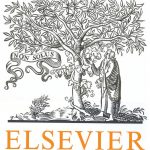
ترجمه مقاله نقش ضروری ارتباطات 6G با چشم انداز صنعت 4.0
- مبلغ: ۸۶,۰۰۰ تومان
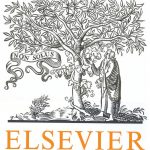
ترجمه مقاله پایداری توسعه شهری، تعدیل ساختار صنعتی و کارایی کاربری زمین
- مبلغ: ۹۱,۰۰۰ تومان
Abstract
Cogeneration is the simultaneous generation of electricity and useful heat with the aim of exploiting more efficiently the energy stored in the fuel. Cogeneration is, however, a complex process that encompasses a great amount of sub-systems and variables. This fact makes it very difficult to obtain an analytical model of the whole plant, and therefore providing a mechanism or a methodology able to optimize the global behavior. This paper proposes a neuro-genetic strategy for modeling and optimizing a cogeneration process of a real industrial plant. Firstly, the modeling of the process is carried out by means of several interconnected neural networks where, each neural network deals with a particular sub-system of the plant. Next, the obtained models are used by a genetic algorithm, which solves a multiobjective optimization problem of the plant, where the goal is to minimize the fuel consumption and maximize both the generated electricity and the use of the heat. The proposed approach is evaluated with data of a real cogeneration plant collected over a one-year period. Obtained results show not only that the modeling of the plant is correct but also that the optimization increases significantly the efficiency of the cogeneration plant.
5. Conclusion and future work
We have presented a neuro-genetic approach for both modeling and optimizing a complete real CHP plant. The modeling is accomplished by means of interconnected neural networks, where each network deals with one sub-system of the plant. Obtained error rates in the experiments show a satisfactory modeling for both training and test data. Next,multiobjective evolutionary algorithms use the NNs as black box functions for finding optimal operation schedules. Different evolutionary algorithms have been analyzed and obtained results have revealed that ESPEA is the most suitable algorithm to solve the problem. Unlike other similar approaches proposed in the literature, our work addresses the CHP plant problem in a wider and more general way: (1) all the sub-systems of the CHP process are considered; (2) both the modeling and the optimization are carried out using soft computing approaches; (3) only real values fromthephysicalplant areusedinthe work;(4)the optimization has been carried out as a multiobjective problem; and (5) the optimization algorithm is fast enough to allow an on-line optimization of the plant. Our findings can spur further research into robust optimization formulations across uncertainty modeling in parameter configurations for cogeneration plants. Our future research focuses on combining different preferences function with our approach, such as tradeoff based techniques [75–78]. A special emphasis is on multiobjective algorithms that employ a cone-based domination technique [79,80]. Cone-based domination have shown to be a powerful tool in preference modeling [81–83]. Further soft computing techniques besides evolutionary algorithms, such as artificial immune systems [84] or infeasibility driven optimization [85] or techniques from [86,87] may also be applied to solve the optimization problem proposed in this paper. Another interesting approach would be to approximate the activation function used in our study, and to have a mathematical formulation of our optimization problem. Fast exact algorithms, like Newton-based techniques [88], could then be applied to solve this optimization problem.