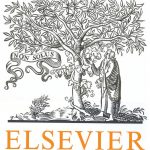
ترجمه مقاله نقش ضروری ارتباطات 6G با چشم انداز صنعت 4.0
- مبلغ: ۸۶,۰۰۰ تومان
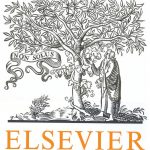
ترجمه مقاله پایداری توسعه شهری، تعدیل ساختار صنعتی و کارایی کاربری زمین
- مبلغ: ۹۱,۰۰۰ تومان
ABSTRACT
The fusion of soft computing methods such as neural networks and evolutionary algorithms have given a very promising performance for time series prediction problems. In order to fully harness their strengths for wider impact, effective real-world implementation of prediction systems must incorporate the use of innovative technologies such as mobile computing. Recently, co-evolutionary algorithms have shown to be very promising for training neural networks for time series prediction. Cooperative coevolution decomposes a problem into subcomponents that are evolved in isolation and cooperation typically involves fitness evaluation. The challenge has been in developing effective subcomponent decomposition methods for different neural network architectures. In this paper, we evaluate the performance of two problem decomposition methods for training feedforward and recurrent neural networks for chaotic time series problems.We further apply them for financial prediction problems selected from the NASDAQ stock exchange. We highlight the challenges in real-time implementation and present a mobile application framework for financial time series prediction. The results, in general, show that recurrent neural networks have better generalisation ability when compared to feedforward networks for real-world time series problems.
6. Conclusions and future work
We presented a comparisonoffeedforward and recurrentneural networks performance for time series prediction where cooperative neuro-evolution was used as the training algorithm. We used two different problem decomposition methods in cooperative neuro-evolution. The results show that the recurrent neural network architecture with neuron level decomposition gave the best results across the different problems. A mobile application framework was also presented that has the potential to efficiently synchronise the prediction system with smartphones taking into account the challenges of over-heating and power consumption. It was highlighted that security issues need to be death with in the implementation of biometric features in the mobile application. This can give potential investors real-time information on market behaviour which is useful in making investments. In future research, it would be interesting to apply the prediction method to different financial problems that deal with foreign exchange rates, interest rates, and dividend rates. Multi-variate time series can also be used to further improve the prediction. Furthermore, machine learning approaches such as transfer learning could be used to exploit fundamental knowledge in different markets that generate the time series.