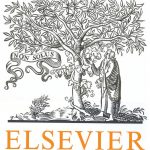
ترجمه مقاله نقش ضروری ارتباطات 6G با چشم انداز صنعت 4.0
- مبلغ: ۸۶,۰۰۰ تومان
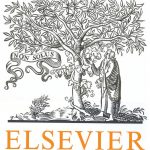
ترجمه مقاله پایداری توسعه شهری، تعدیل ساختار صنعتی و کارایی کاربری زمین
- مبلغ: ۹۱,۰۰۰ تومان
Abstract
Recent data-driven soft sensors often use multiple adaptive mechanisms to cope with non-stationary environments. These mechanisms are usually deployed in a prescribed order which does not change. In this work we use real world data from the process industry to compare deploying adaptive mechanisms in a fixed manner to deploying them in a flexible way, which results in varying adaptation sequences. We demonstrate that flexible deployment of available adaptive methods coupled with techniques such as cross-validatory selection and retrospective model correction can benefit the predictive accuracy over time. As a vehicle for this study, we use a soft-sensor for batch processes based on an adaptive ensemble method which employs several adaptive mechanisms to react to the changes in data.
6. Discussion and conclusions
The core aim of this paper was to investigate the behaviour of a data-driven soft sensor with multiple adaptive mechanisms. We have conducted experiments on 3 real datasets from the process industry, exhibiting different properties and different rates of change. We observe that in most of the cases, using multiple AMs is better than using only one, even the most suitable AM for the dataset. This is true for Catalyst and Oxidizer datasets. Here, having the possibility to deploy AMs which are rarely the best, brings an improvement to the predictive accuracy of the soft sensor. For the Drier dataset, using just AM1 (batch learning with no forgetting) provides good results. This is related to the lack of change in dataset – hence the AMs which apply stronger adaptation to the models are not needed and overcomplicate the model. Similarly, using SABLE with flexible AM deployment strategies provided better results for most of the cases. Here the comparison is mostly between Joint (deploying all of the available AMs on the same batch) and flexible configurations. We have seen that choosing the AM which minimizes the error for the next batch (Optimal configuration) provides better results than Joint. As shown in Bakirov et al. (2016), XVSelect (using crossvalidatory selection based on the last available batch) achieves quite high predictive accuracy levels. This can be further improved by retrospective AM correction mechanism. Generally XVSelect strategy with correction provides the best predictive accuracy in most of the cases. Considering all of the above, we can conclude that in a batch learning scenario, using multiple adaptive mechanisms with flexible deployment order which is identified using cross-validatory selection together with the application of retrospective model correction provides significantly better results than simple retraining, deployment of separate AMs and their joint deployment on every batch.